Authors: Dmitry Victorovich Malakhova – 1, Boris Vasilievich Zlatanov – 2
1 – Joint Stock Company “National Center for Space Research and Technology”, Almaty, 050010, Kazakhstan.
2 – Institute of Zoology, Ministry of Education and Sciences, Almaty, 050060, Kazakhstan.
Abstract
Since the earliest times, the problem of the locust has been a very serious one for agriculture and food production in particular. Devastating locust plagues are known from the Old and the New World where locust swarms terrified the earliest settlement and towns, leaving not a single green branch on the fields.
Ways to combat locust plagues were often ineffective or extremely expensive due to a lack of information on the exact location of the newly hatched locusts; and the lack of an accurate assessment of the number of locusts in currently forming swarms. Rapidly developing GIS applications, especially as part of environmental modelling, appear to be a good means of forecasting a locust plague, being based on accurate ground observation and expert knowledge of the biology and ecology of the locust species. This paper represents an example of extended ecological modelling for the nesting conditions of Dociostaurus maroccanus, a well-known gregarious acridid species, with emphasis on the explanation of key environmental variables as revealed by the model.
To download full version of article in PDF format – open in new tab
Introduction
Dating the co-evolution between bees and beetle triungulins (Coleoptera: Cleridae) to the mid-Cretaceous
Dociostaurus maroccanus (Moroccan locust, DMA) is a thermophilous and xerophilous acridid species inhabiting open, well-lit areas (Bounechada, 2007). The natural area of Dociostaurus maroccanus comprises the Mediterranean (sensu lato) from the Atlantic Ocean islands (Madeira, Canary Islands) in the west to Afghanistan and Kazakhstan in the east. The area of the species is heavily fragmented. Nesting areas are separated from each other by mountain ridges and large bodies of water (Monar et al., 2009). The species is mainly adapted to valleys and foothills with xerophytic vegetation at an altitude of between 400 and 800 m.a.s.l. (Song, 2011). The current area of the species appears significantly changed due to climatic fluctuations and human activity. Dociostaurus maroccanus represents a serious transboundary problem in Central Asia. Active and permanent breeding foci are located in both sides of several international boundaries. Swarms coming from outside the boundary require to be controlled from both countries. The monitoring of swarm migration is an important measure to prevent economic losses (Bounechada, 2007). The Moroccan locust is one of the most dangerous pests and on an annual basis causes significant damage to agriculture around the world. In Kazakhstan, the zone of mass reproduction for the Moroccan locust is located in the southern part of the country. Cross-border flights of the locust swarms occur mainly between South Kazakhstan and the neighboring countries, Uzbekistan and Kyrgyzstan (Azhbenov et al., 2015).
An appropriate approach to understanding the pattern of locust outbreaks and to developing adequate monitoring and preventative measures is ecological modelling of the vulnerable stages of the life cycle of the locust.
Ecological modelling involves the outlining of the key environmental variables and their ranges; evaluation of the spatial and temporal distribution of the variables; and provides a basis for making reasonable decisions on how the risk of outbreaks may be counteracted.
The methods and approaches of both the Species Distribution Model (SDM) and the Ecological Niche Model (ENM) represent a rapidly developing scientific trend with a particular viewpoint (Franklin, 2010; Graham & Hijmans, 2006). An important benefit of ecological models designed to mitigate the impact of locust swarms is that they can help reduce the overuse of chemical insecticides. Generating maps that express the relative risks of outbreak events might facilitate more reasonable, effective and environmentally sustainable use of chemical insecticides, controls and integrated pest management (Aragón, Coca-Abia, Llorente, & Lobo, 2012). Success of the modeling procedure depends upon several factors. The most important is the collection of a set of ground data which is as complete as possible and which documents the known species distribution. A limited or incomplete set of ground data will certainly affect the cognitive and analytic value of the resulting model. In general, there are four classes of factors, which determine the areas in which a species may be found (Soberón & Peterson, 2005):
- Abiotic conditions, including aspects of climate, physical environment, edaphic conditions, etc., that impose physiological limits on a species’ ability to persist in an area.
- The regions that are accessible to dispersal by the species from some original area. This factor is extremely useful in distinguishing a species’ actual distribution from its potential distribution, based on landscape configuration and the species’ dispersal abilities.
- Biotic factors: the set of interactions with other species that modify the species’ ability to maintain populations. These interactions can be either positive (e.g., mutualists such as seed dispersers, pollinators, etc.) or negative (e.g., competitors, predators, diseases). By limiting or enhancing population processes, interactions can obviously affect distributions.
- The evolutionary capacity of populations of the species to adapt to new conditions. This factor, usually reserved from analyses or assumed negligible, is nevertheless an additional and important consideration in outlining the distributional possibilities of species. In theory and in the limited experiments carried out to date, the effects of evolution in niche parameters over short periods of time appear minor.
The first class (the set of abiotic variables) is currently available for analysis, being obtained from several sources (see Material and methods) and is widely used in modeling applications. The second class may be easily derived from the analysis of the final model; whereas the third and the fourth classes are much harder to formalize and include the existing methods of ENM.
For the purposes of our study, we follow the theory of Hutchinson (1957), who formalized the niche concept as an attribute of the species rather than the environment. Hutchinson’s niche exists in a space (n-dimensional hypercube) occupied by biotic and abiotic environmental variables, some of which represent the limits of species viability. With Hutchinson’s formal definition of the ecological niche, it is possible to describe and quantitatively estimate all types of ecological niche, although with differing degrees of accuracy. A niche-based model represents an approximation of a species’ ecological niche in the environmental dimensions under examination. A species’ fundamental niche consists of the set of all conditions that allow for its long-term survival; whereas its realized niche is that subset of the fundamental niche that it actually occupies (Hutchinson, 1957). The species’ realized niche may be smaller than its fundamental niche, due to human influence; biotic interactions (e.g., inter-specific competition, predation); or geographic barriers that have hindered dispersal and colonization. Geographic areas, depicted in terms of a model as favorable for the species, represent rather the fundamental niche; and areas where the species is actually present constitute the realized niche.
It is necessary to make a clear distinction between the Species Distribution Model (SDM) and the Ecological Niche Model (ENM). The SDM usually does not require deep analysis of the variables and simply provides a map of a suitable habitat for the species. Usually, also, the modeler uses a predefined (or a “customized”) set of variables, based upon the generally accepted knowledge of the biology of the species (Beaumont et al., 2005); and, in general, this may not reflect the peculiarities of the relationship between the animal and the environment for the given species. SDM shows itself to be a purely statistical approach that bears a weak relationship to the natural peculiarities of the species.
The ENM procedure is carried out basically in the same way as SDM but involves an extended set of variables; and, what is more important, aims to provide a detailed analysis of each variable’s importance to and influence on the animal, over and above simple habitat-suitability map development. In brief, the ENM deals with the fundamental niche; and SDM is focused on the realized niche (Peterson & Soberón, 2012). The main question associated with the SDM procedure is: “Has the map been developed in good accordance with the species’ area?”. If the answer is “yes”, then the goal of the modeling procedure has been accomplished. By contrast, ENM answers the question: “Which key variables may affect the species’ wellbeing and how do they do so?”. It also describes the geographical consistency of the developed map. Prediction of species outbreaks or species invasion into unoccupied areas is related to an understanding of the fundamental niche of a given species and falls into the ENM process; whereas the mechanistic, SDM-based approach may give rise to dubious interpretations.
Materials and methods
439 ground points of documented nesting sites of D. maroccanus were obtained from published and own data (Fig. 1). The modeling procedure, which was used within the current model, was basically designed and tested with a number of other species, (Dujsebayeva & Malakhov, 2017; Malakhov & Chirikova, 2018; Malakhov, Tsychueva, & Vitkovskaya, 2017; Malakhov et al., 2018). The following climatic data sets were applied to develop the current model: WorldClim (monthly temperatures and precipitation, solar radiation, vapor pressure); BioClim (a set of variables derived from WorldClim and meteorological stations) (http:/www.worldclim.org); Global Potential Evapo – Transpiration http:/www.cgiar- csi.org/data/globalaridity- and-pet-database); Digital Elevation Model and its derivatives like exposition, slope, curvature etc., computed with ArcGIS functions. WorldClim and BioCLIM datasets and their applications in ecological modeling are well described (Booth et al., 2013; Hijmans et al., 2005; Houlder et al., 2001). Global-Pet is an index combining transpiration and evaporation (Allen et al., 1998; UNEP (United Nations Environment Programme, 1997).
Data on NDVI and Soil Water Index (SWI) were obtained from Copernicus Global Land Service (https://land.copernicus.vgt.vito.be/PDF/portal/Application.html#Home) and averaged monthly for 20 years and 12 years for NDVI and SWI respectively with QGIS functions.
The previously applied modeling process has been modified with the focus on a more accurate selection of key variables and a more precise result. Modifications are concerned with the stage at which key variables are selected. We apply two basic rules to the selection of weighted variables: the rule of control points; and the rule of variable’s normal distribution. We applied three randomly selected sets of control points, each equal to 25% of the total of 439 ground points. Each control set was checked for consistency of points and optimal range of each variable. If the consistency of two or three sets of control points in relation to the optimal range of a given variable is higher than 70%, this variable is considered for further analysis. The next step in the validation of the importance of a variable is to check the value distribution of the variable within the total range of ground points. It can be hypothesized (Beaumont et al., 2005) that variables with normally distributed values may have an important influence on the distribution of the species. Variables that demonstrate skewed distribution may also be relevant. Variables with skewed distributions may be those that do not have a negative value, such as solar radiation; and those that have values between zero and one, such as vegetation indices. Where there is no clear pattern in the distribution histograms for a variable, that variable could be classified as irrelevant. Similarly, where the histogram is normally distributed but is truncated in one or both tails, the variable could also be rejected, as these graphs suggest that the species could tolerate other values of this variable that were not included in the species’ climatic envelope. This may occur if the distribution records for a species do not cover its entire geographic range. (Beaumont et al., 2005). Variables passing the “normality” check were used as part of a final list of key variables in order to calculate the model. The list of key variables with the optimal ranges for each variable is represented in Table 1.
The graphical representation of the model was computed with the Weighted Sum function in ArcGIS (Fig. 2). The model’s accuracy was tested with ground data on egg- pods collected during 2018 in Southern Kazakhstan.
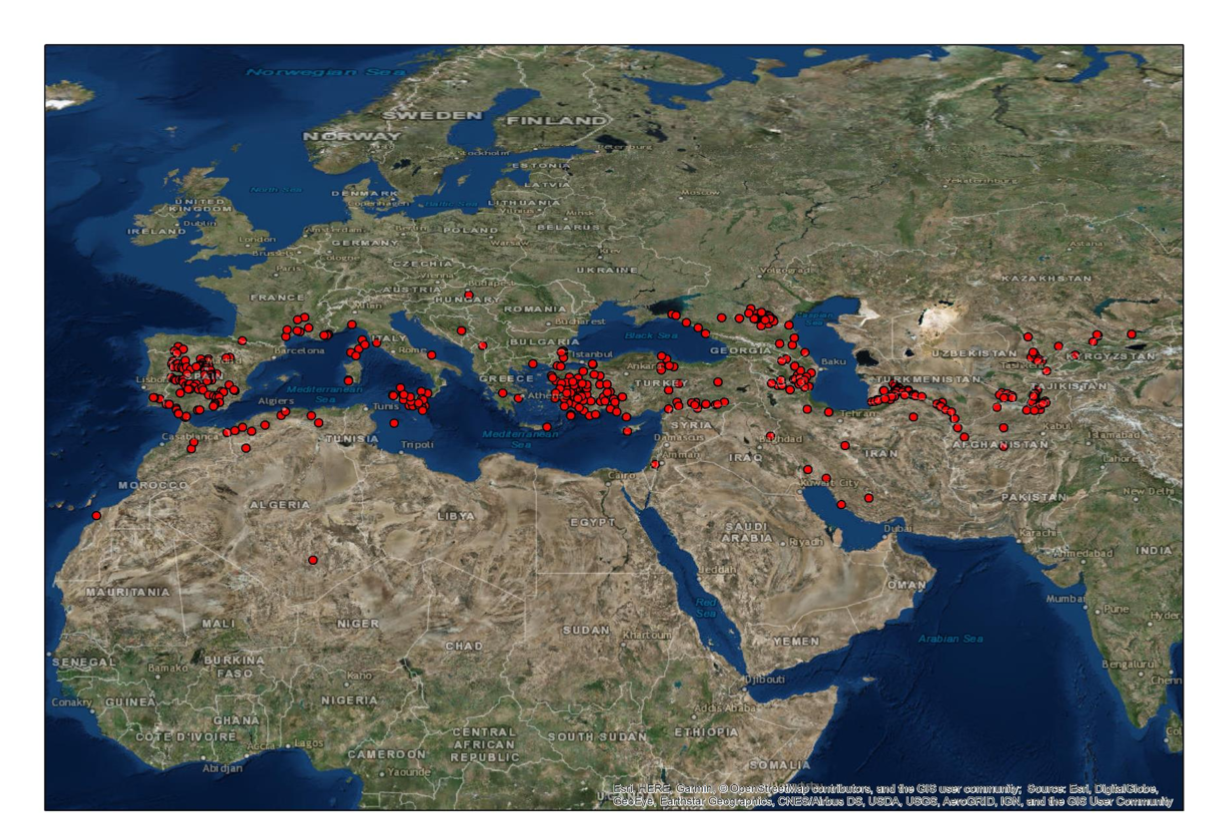
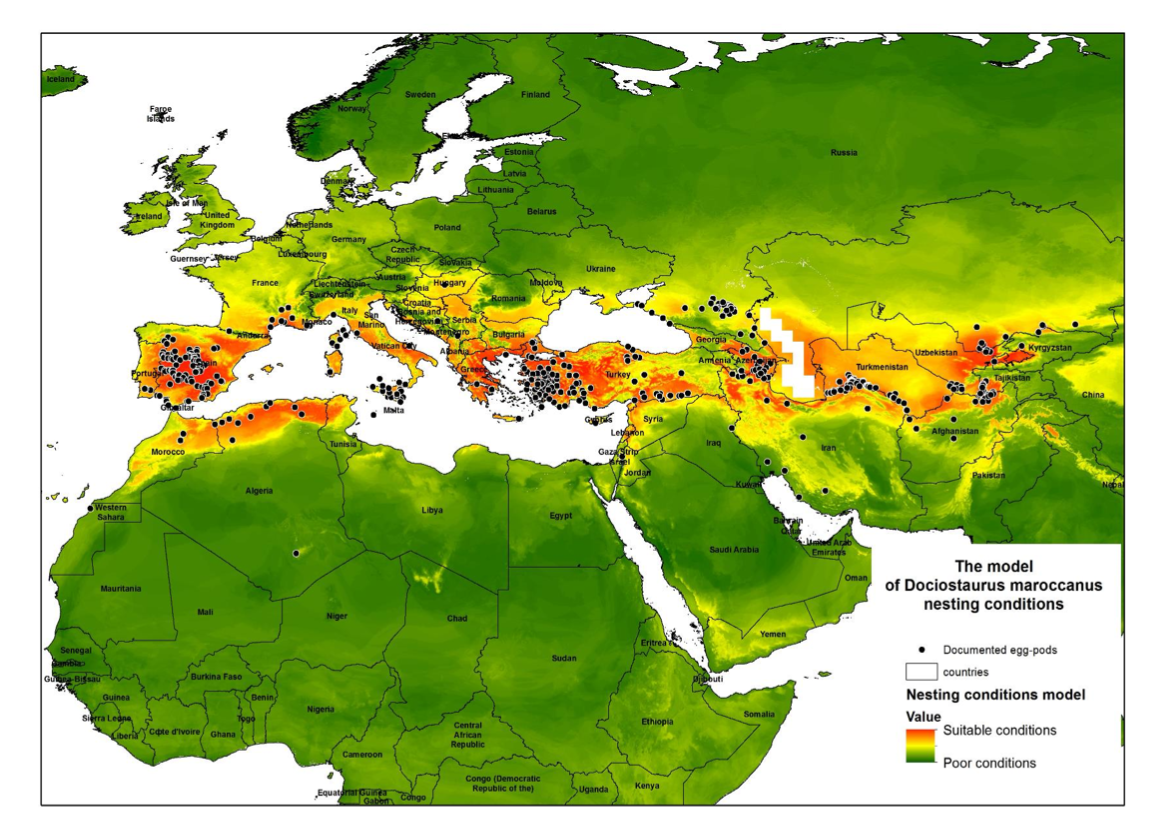
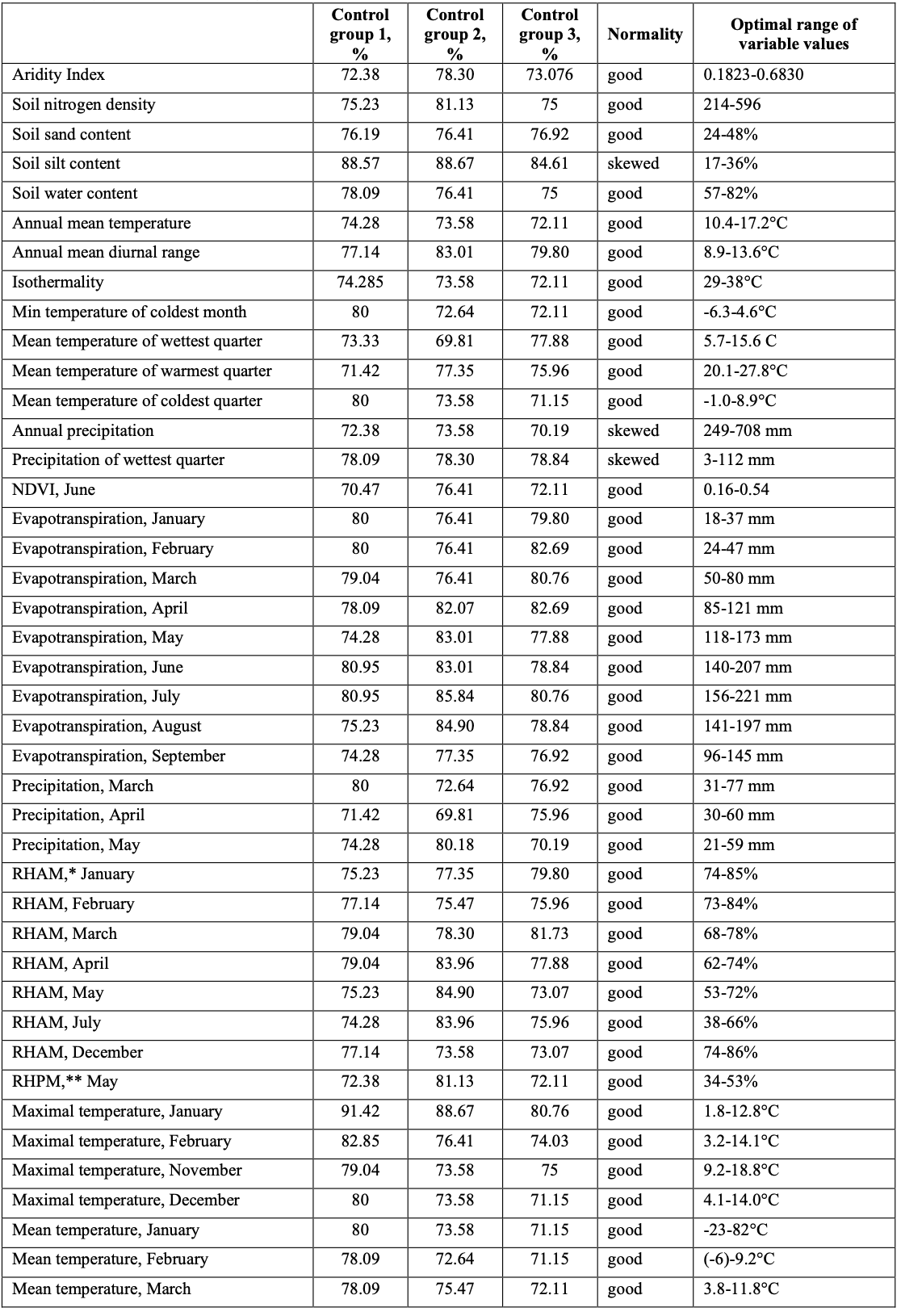
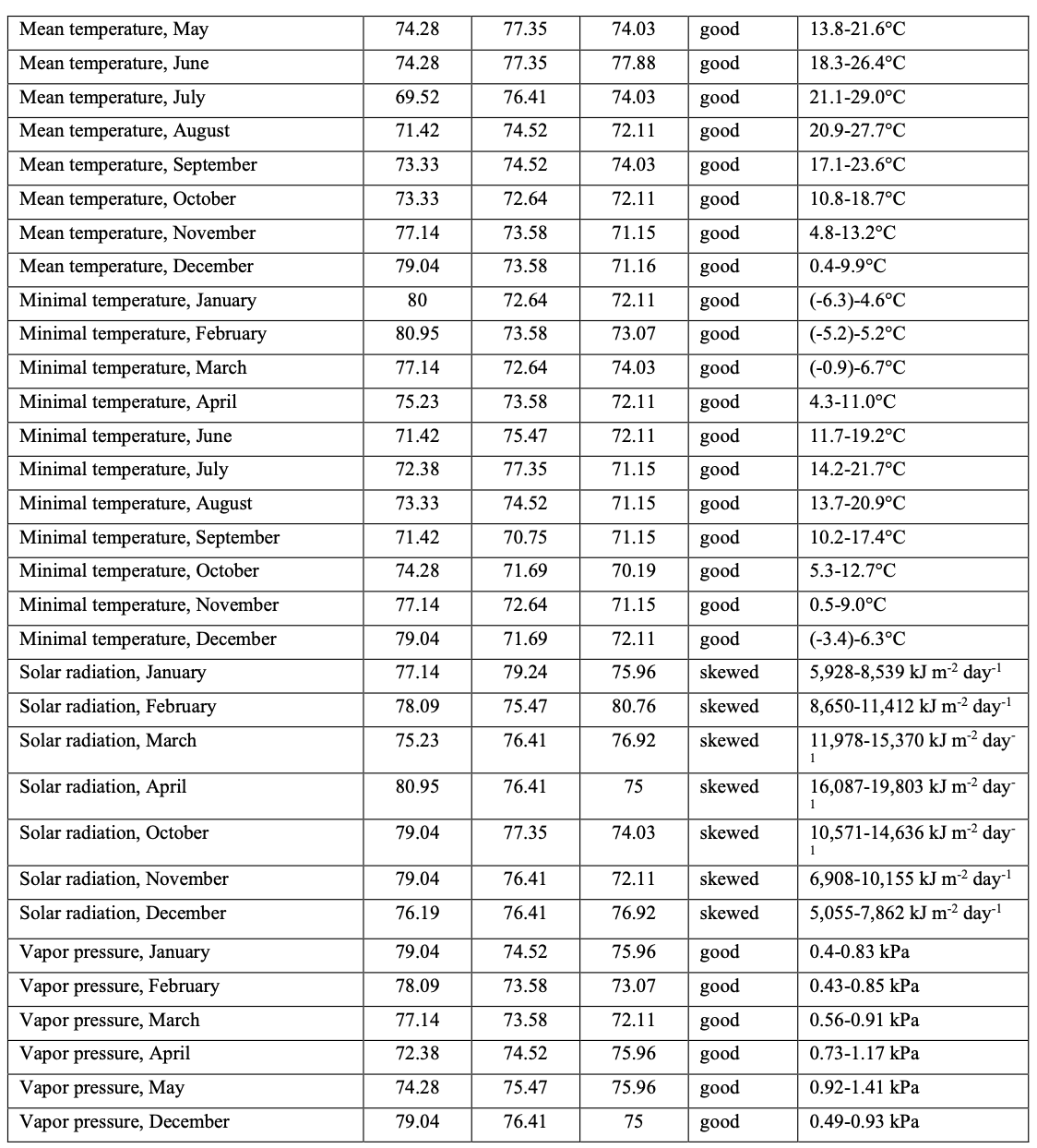
Results
The model developed (Fig. 2) reveals good spatial agreement with the currently known distribution of the nesting areas of D. maroccanus. The model identifies the existence of favorable conditions round almost all of the Mediterranean Sea, with main foci located on the Iberian Peninsula and in North Africa; southern Europe and Asia Minor; and the Caucasus and North Caucasus. The Caucasian hotspot extends eastward via Northern Iran and almost meets the Central Asian hotspot. The latter comprises North Afghanistan; the southern territories of Tajikistan; north-east Uzbekistan; and extends as far as south Kazakhstan.
Analysis of the list of key variables shows the importance of wetness-related variables (precipitation, evapotranspiration, relative air humidity) and temperature, along with a number of other variables. 43 of 74 key variables (58%) describe winter and spring conditions. The ratio of summer to autumn variables is 30%. The remaining variables (12%) refer either to annual data or to non-climatic (soil properties) data (Fig. 3).
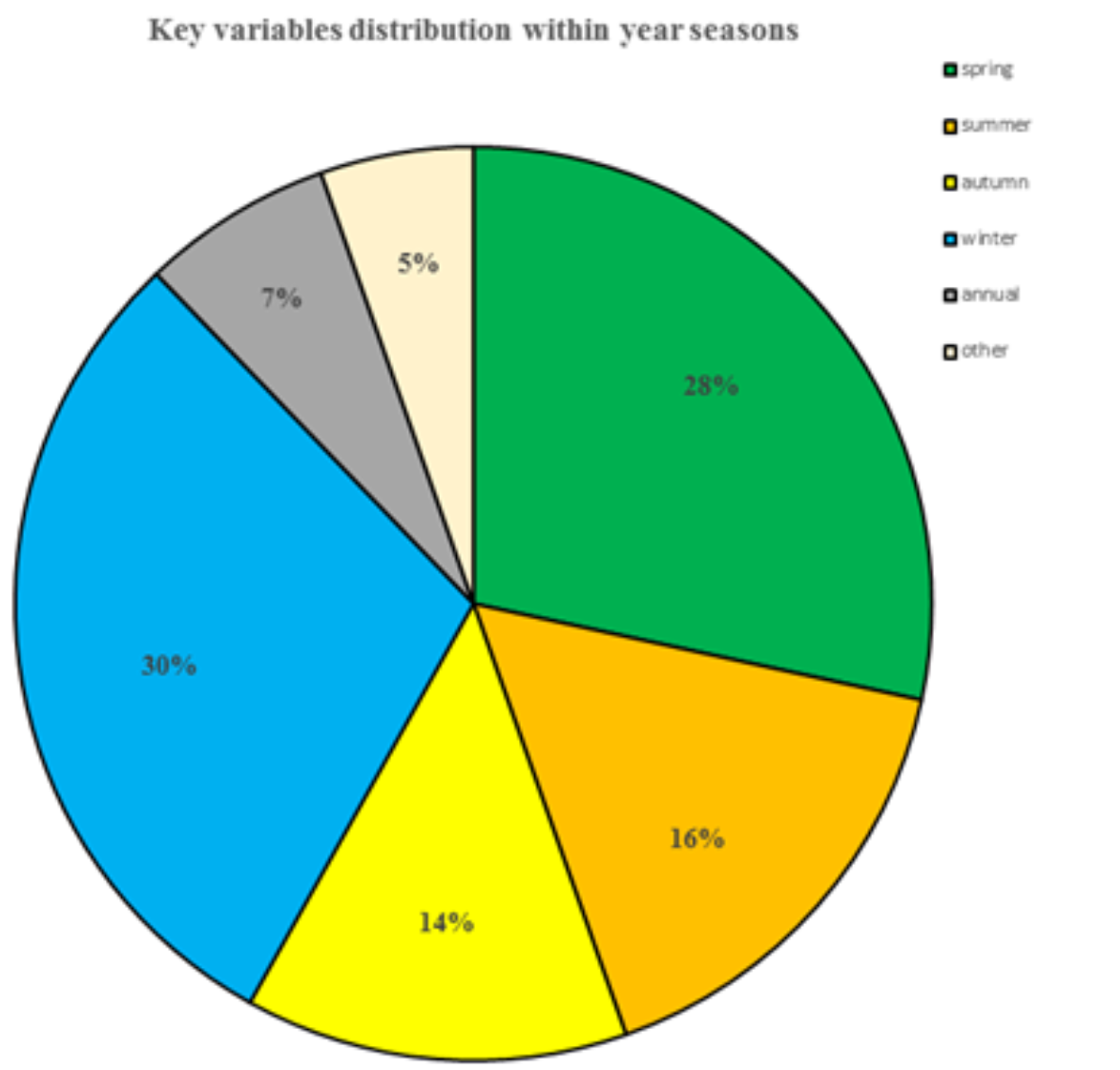
This correlates well with the known data on the physiology and life cycle of the species, in which the most important and vulnerable stages occur during the embryogenesis and early development of the nymph. Data used for the model validation (181 ground-points) were not included in the modeling procedure, and the distribution of egg-pods in 2018 showed almost perfect consistency with the model. The range of values for the model was divided into ten classes (from zero to 100% suitability with a step of 10%) and the distribution of ground data was calculated for each model class (Fig. 4). The validation procedure revealed that no locality falls beneath 50% suitability in terms of the model, and more than half of recently documented localities (94 out of 181) occupy the 90-100% range of suitability as measured by the model.
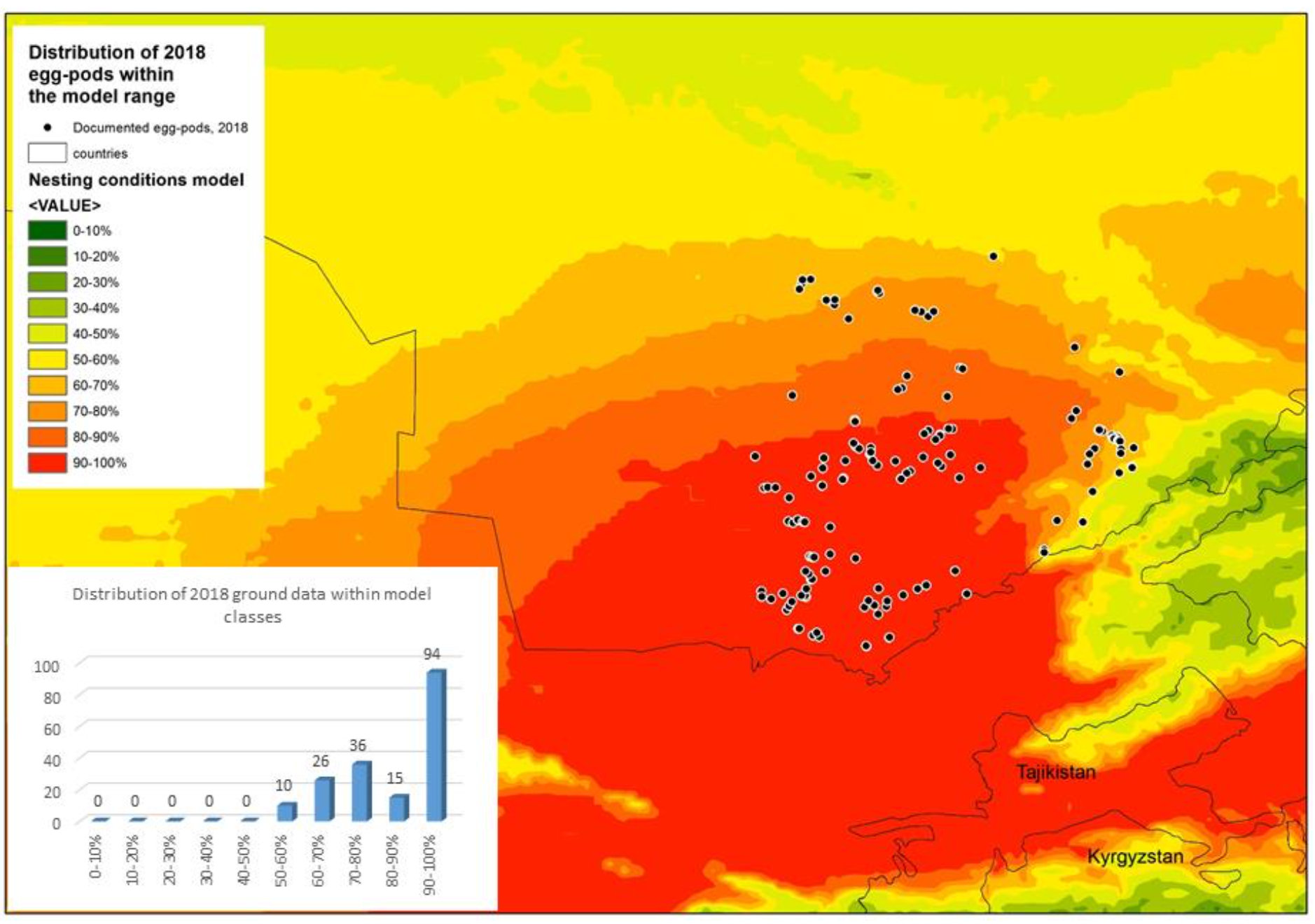
The particular meaning of the key variables is discussed below.
Discussion
Relationships of abiotic variables
Solar radiation at the Earth’s surface is the principal and fundamental energy for many physical, chemical and biological processes, such as crop growth and plant photosynthesis; and it is also an essential and important variable within many simulation-model studies, such as agriculture, environment, hydrology, meteorology, and ecology (Chen & Li, 2014). Daily solar irradiance at the earth’s surface is a fundamental driving variable for simulation of the ecosystem in terms of carbon, water, and energy fluxes on the local, regional, and global scales (Winslow et al., 2001). Solar radiation partially regulates relative air humidity (Swartman & Ogunlade, 1967). Air temperature correlates with relative humidity (Lawrence, 2005); and solar radiation influences precipitation and air temperature (Bindi & Miglietta, 1991; Ituen et al., 2012; Winslow et al., 2001). Evaporation is related to incoming solar radiation (Beard & Gainer, 1969). The surface temperature may be derived from known solar radiation (Daut et al., 2012). Evaporation may be estimated using Dalton’s equation (Beard & Gainer, 1969). In turn, the evapotranspiration value is related to evaporation (Allen et al., 1998). The known evapotranspiration and precipitation values produce another very important variable (especially for ectotherm animals and vegetation) – Aridity Index (UNEP (United Nations Environment Programme), 1997). There are known well-established correlations of air and soil temperatures (Ahmad & Rasul, 2008; Islam et al., 2015).
It is obvious that abiotic variables, such as air temperature, soil moisture, and air humidity, solar radiation and evaporation do not exist separately, as they all represent different aspects of the entire process of the energy balance. Important for the ENM procedure is the ability to estimate the range of directly unavailable variables from ranges, as provided by the model as a result of the variables which are processed. Each variable value may be calculated with a more or less simple equation when the value of the related variable is known.
Notes on Dociostarus maroccanus life-cycle
The life-cycle of D. maroccanus is considerably well studied, as the species attracts the attention of many researchers (Bey-Bienko & Mischenko, 1951; Latchininsky et al., 2002; Predtechensky et al., 1935; Syazov, 1912; Tsyplenkov, 1970). Uvarov (1955) described the life-cycle of D. maroccanus as follows:
This species is connected with a single vegetation type, that of dry steppe or semidesert with abundant spring ephemeres, of which Poa bulbosa is the most typical. The seasonal development of this type of vegetation is characterized by very rapid sprouting after the first spring rains, a short period of lush growth, and almost complete dying off at the end of spring so that the spring and summer aspects of the same area appear totally different. Hatching from hibernated eggs occurs after the first warm spring rains so that the hoppers find abundant fresh food. By the time the adults appear, the ephemeral vegetation is dried up and locusts tend to concentrate in patches of taller perennial vegetation which provide food and shelter for adults. Egg-laying, however, takes place not among the latter but on the bare patches where the ephemeres grew earlier, and it is reasonable that the type of soil required by these plants is also preferred by the Moroccan Locust (p. 23- 25).
As much as two-thirds of nesting sites fall within only two climatic zones accordingly to Köppen-Geiger’s classification (Kottek et al., 2006) (Fig. 5).
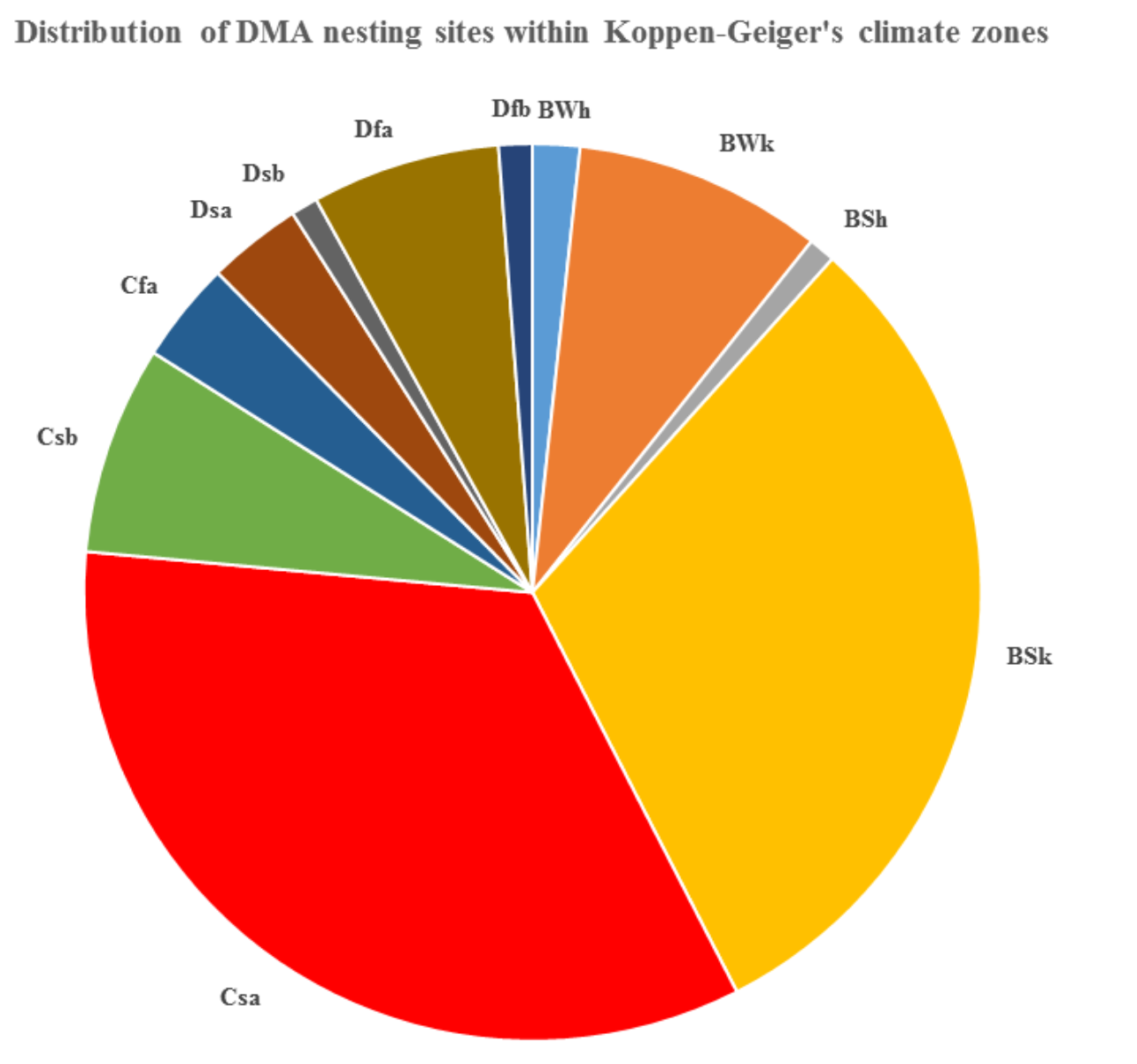
The zones where most of the nesting sites are located (BSk and Csa) are characterized as follows:
BSk – Arid/Steppe/Cold; 𝐶sa
Summer/Hot Summer.
BWk, Dfa and Csb represent the most important zones;
and the rest make up the remaining third. Bwk is
Arid/Desert/Cold, Dfa is Cold/Without dry summer/Hot
summer, and Csb is Temperate/Dry summer/Warm
Summer. It is obvious that DMA’s nesting sites cover
quite a variety of climatic conditions. All but one of the
zones are characterized by a lack of water during some
seasons or indeed throughout the entire year.
The density of the surface soil layer is of great importance for the locust, as the species inhabits intact, considerably wet and hard soils (at least, the relatively high water content and the soil density are confirmed by the model data, see Table 1) and disappears if those lands are plowed (Bey-Bienko & Mischenko, 1951). The number of egg-pods per square meter varies from about 1500 to a maximum density of 6000. Each egg-pod contains 18-42 eggs. Egg-pods are laid in dense, mainly stony foothill soils (Syazov, 1912; Yakhontov, 1964). Usually, a single female provides two egg-pods (Quesada-Moraga & Santiago-Álvarez, 2001).
The distribution of the egg-pods has a markedly “spotted” character (Bey-Bienko & Mischenko, 1951; Syazov, 1912; Uvarov, 1955). In Foggia’s flat land, D. maroccanus egg-pods are concentrated within small areas of 1-2 meters in diameter, separated from each other by small clumps or strips of lush vegetation, unevenly distributed over several square kilometers of hard and bare soils (Santiago‐Alvarez et al., 2003). Regarding micro relief, the egg-pods are found to be laid on drier hummocks, whereas nymphs and adults feed within moist depressions (Benhalima et al., 1984). The hatchling may live for 1-2 weeks in northern exposures. (Bey-Bienko & Mischenko, 1951). The Moroccan locust is characterized, as all desert locusts are, with an early hatchling period usually from the end of March in southern areas or the first half of April in northernmost areas (Bey-Bienko & Mischenko, 1951). The hatch usually takes place from February to April; and the nymph’s development has a synchronous pattern (Bey- Bienko & Mischenko, 1951; Monar et al., 2009; Popova & Popov, 2009; Syazov, 1912). Nymph development is quite rapid, lasting no more than 25-35 days. The adult stage actively migrates from the hatchling sites to humid depressions where the adults find a sufficient amount of food (Popova & Popov, 2009). The breeding season starts in May; and by mid-summer the adults are dying out (Monar et al., 2009). The egg’s embryo and hibernation stages are thus the most important and vulnerable parts of the species’ life-cycle.
Thermal issues
The surface and subsurface soil temperature may be estimated with appropriate accuracy from the known air temperature (Ahmad & Rasul, 2008; Islam et al., 2015). Here, we have calculated the estimated soil temperature within the optimal range of key air-temperature ranges obtained from the modeling procedure (Table 2).
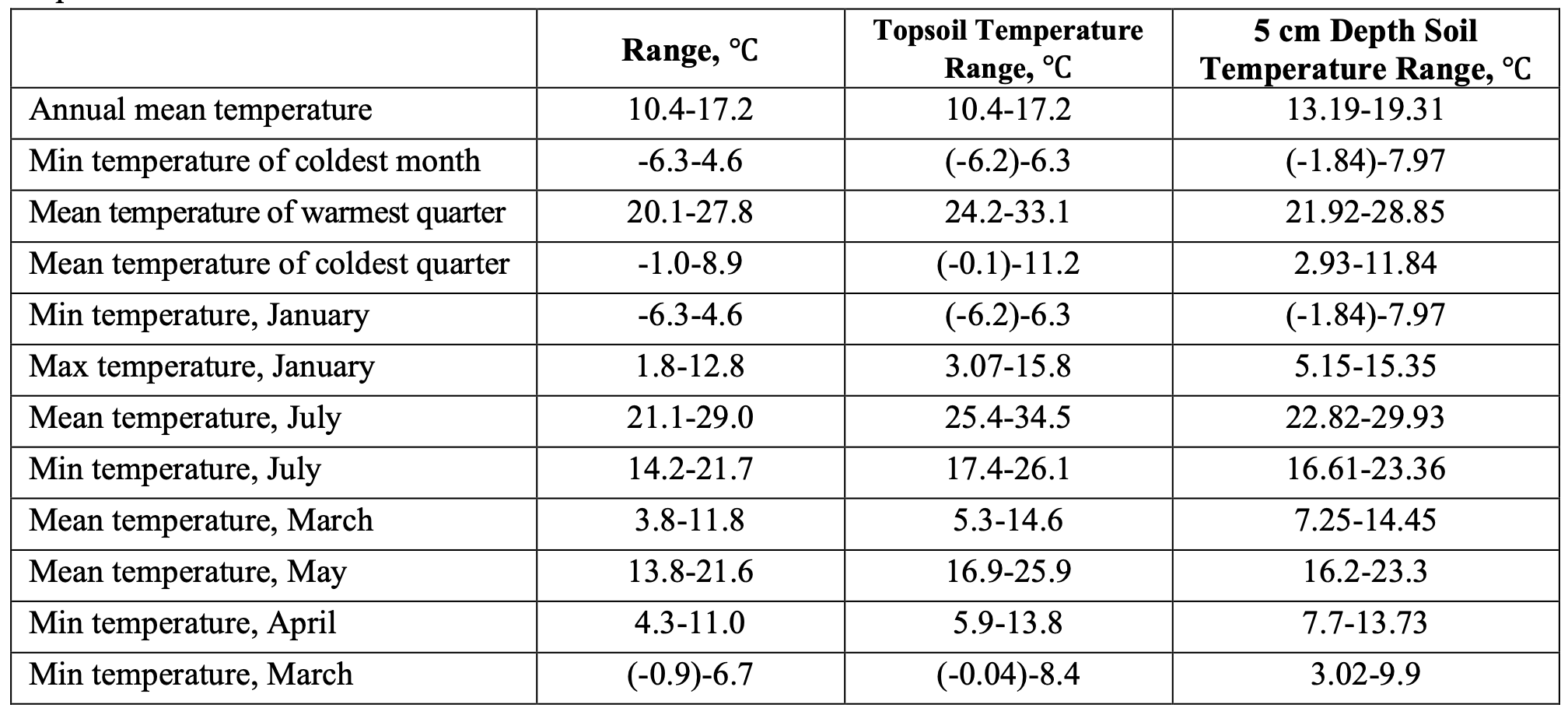
The embryogenesis of DMA is interrupted when the ambient temperature exceeds 24.7 °C. The mean air temperature in August (Table 1) is one of the possible regulators switching normal embryogenesis to diapause. The post-diapause-development thermal threshold is below 16 °C. Under some favorable circumstances, normal embryogenesis may start again as early as January (Table 2), when the temperature of the soil at the 5 cm depth rises close to 16 °C. Embryonic development follows its normal course when the air and surface temperatures are below 25 °C. (Arias, Jiménez, & Pérez, 1997; Santiago‐Alvarez et al., 2003). However, nymph development takes place at higher temperatures than egg development. The fact that locusts select temperatures near 38 °C in the thermal gradient, despite reduced nutrient utilization efficiency, suggests that the benefits outweighed the costs of doing so (Miller et al., 2009). As Table 2 shows, the optimal range for the mean air temperature of the warmest quarter varies between 20-28 °C. This range is good for the development of nymph stages, bearing in mind that the temperature of the topsoil level is higher than the ambient air temperature; and nymphs benefit from peculiarities of heat distribution related to microrelief via behavioral thermoregulation.
The air temperature is still of great importance for adults. Grasshoppers exposed to high air temperature are able through a physiological response to maintain their internal temperature within the range necessary to avoid thermal death (under 48 °C). Ventilation rate primarily serves the function of evaporative cooling. Air sacs which are present in the tracheal system of grasshoppers seem likely sites for evaporation and also function as thermoregulatory organs (Prange, 1990). The locust does not regulate its thoracic temperature during flight, and as a result the thoracic temperature of a flying locust generally exceeds the ambient temperature by 5-8 °C. The minimum thoracic temperature at which flight is initiated is 24 °C; and the maximum temperature for flight muscle contraction is 42 °C (Foster & Robertson, 1992). The upper limit of mean temperature in June, according to the model, is 29 °C, and this temperature presents no obstacle to flight.
In some acridid species, the autumn stage of the embryogenesis requires a relatively high temperature and a moderate degree of moisture (Bey-Bienko & Mischenko, 1951). Data from the model confirms that the mean autumn temperature varies between 4.8 and 27.7 °C, remaining quite high. The only key variable related to autumn humidity is evapotranspiration in September. This varies considerably in the high range of 96-145 mm/month. Both temperature and evapotranspiration values appear to be in accordance with the locust’s requirements as far as autumn embryogenesis is concerned.
The nymphs of the Asiatic locust (L. migratoria) may withstand short-term cooling up to minus five degrees, and they remain active in 10-55 °C range. The locusts’ body temperature is regulated mainly by solar radiation; whereas air humidity is on the other hand related to the level of water content in body tissues (Tsyplenkov, 1970). Although we did not find direct data on DMA, the temperature conditions which it requires should be similar to those for other acridid species inhabiting desert or semi-desert areas.
Moisture-related factors
A rainfall level of 80-110 mm in winter and early spring ensures the level of soil moisture required for post- diapause development in D. maroccanus eggs. Of all the abiotic variables, precipitation appears to be the most important for DMA, with an optimal range of about 100 mm in terms of total spring precipitation. In Spain in particular, the optimal precipitation value during late winter and early summer is 80-100 (Santiago‐Alvarez et al., 2003). Outbreaks are controlled most obviously by moisture levels in Spring rather than by other factors (Bey-Bienko & Mischenko, 1951; Popova & Popov, 2009; Predtechensky et al., 1935; Uvarov, 1955). The optimal annual precipitation range for DMA is 300-500 mm (Predtechensky et al., 1935). Our data as provided by the model (Table 1), reveals that the total amount of spring precipitation is about 80-190 mm; and annual precipitation is about 249-708 mm. Key variables include Spring precipitation (precipitation during March and April), where the amount (130mm) is the same for both months. This period is indicated as the hatchling period for D. maroccanus. Precipitation of a given diapason provides the optimal soil-moisture conditions for egg survival. More intensive precipitation leads to embryonic development being retarded and the hatchling period being overextended. If the soil is extremely wet, egg-pods may die out (Monar et al., 2009; Popova & Popov, 2009). In May, a noticeable factor is the importance of air humidity both ante- and post meridiem. This is when the breeding season should start. The air moisture needs to be quite high (more than 70% in the morning and more than 50% in the afternoon). This may well indicate that moisture conditions are being sustained at a level necessary to maintain the required water content in the locust’s tissues, as well as, in all probability, preventing water loss in the organism due to breeding activity.
Vegetation
Precipitation during the late spring is of importance to the growth of vegetation. Adult Moroccan Locusts live twice as long if green vegetation cover persists during the summer. The overall humidity of the warm seasons (spring and summer) is indicated by the “evapotranspiration”, and “relative air humidity” variables. These are obviously related to vegetation being sustained at a level which will provide enough energy for the breeding and egg-laying locusts. The number of eggs laid depends upon the amount of green vegetation consumed by the adult during the season (Dempster, 1963). Years with extremely low values for the vegetation index usually precede years when locust outbreaks occur (Tronin, 2007; Uvarov, 1955).
Fluctuations in the vegetation follow the climatic ones. NDVI (Normalized Difference Vegetation Index), Rouse et al. (1974) was proposed as the ratio of red to near-infrared bands within the satellite imagery. This index is capable of determining the amount of chlorophyll; and thus, to distinguish bare soil from vegetated areas. Analysis of NDVI using the model demonstrates the presence of sparse to moderately dense vegetation cover in the summer months that reflects the food demands of DMA. Unfortunately, it is nearly impossible to derive those plant species the locust prefers from such a simple ratio as NDVI. However, the use of NDVI for the model is justified as a tool to rule out areas with NDVI values which go beyond the optimal ranges shown by the model. The most constant feature of all the habitats of the Moroccan locust is that there is a single type of plant cover (Uvarov, 1955). This species is connected with a vegetation type, that of dry steppe or semidesert with abundant spring ephemeres, of which Poa bulbosa is the most typical. The main habitats of the Moroccan locust are known to be xerophytic biotopes with the prevalence of the thick sedge Carex pachystylis and bulbous bluegrass Poa bulbosa (Kokanova, 2017). Early nymphs and frequently adults feed mainly on Poaceae (Barranco et al., 1997; Chen & Li, 2014; Guisan & Thuiller, 2005; Latchininsky, 2017). The seasonal development of this type of vegetation is characterized by very rapid sprouting after the first spring rains; a short period of lush growth; and almost complete dying off at the end of spring so that the spring and summer aspects of the same area appear totally different. The same type of development characterizes the Moroccan Locust. Although hot and dry weather is comfortable for locust nymphs, it may result in early drying of vegetation, so in a dry summer, the amount of available food may be insufficient for late nymphs and adults (Dempster, 1963). The locust is able to consume its preferred plant species even in dry conditions. The completely dry remnants of Poa bulbosa, Erodium triangulare и Medicago spp were found in the digestive systems of the Locust in July (Ben Halima et al., 1984). If the weather becomes extremely dry, it may trigger a change in the Locust diet. The Locust will switch to water-rich species of vegetation, like Scorzonera pygmaea, that remain green and contain water; but this negatively affects the development of the locust ovaria (Ben Halima et al., 1985). The variables which describe precipitation and humidity in spring and summer are related to the growth of vegetation rather than directly to the locust itself.
Habitat and area shift
The area inhabited by D. maroccanus is somewhat unstable and fluctuating due to its patchy character; and this makes the species sensitive to the climate’s annual fluctuations, which are accentuated in the microclimate of the habitat. Amongst the weather-related factors which control the numbers of D. maroccanus rainfall appears to be particularly important (see above). Usually a plague follows two or more years of deficient rainfall (Uvarov, 1955). The main active nesting foci in Central Asia are as follows (Monar et al., 2009): North Afghanistan; south and north-western Tajikistan; almost the whole of Uzbekistan; south-eastern Turkmenistan; southern Kazakhstan; northern Kyrgyzstan; and some areas in the Caucasus. The number of areas of southern Kazakhstan invaded by DMA nymphs increased by up to 2.4 times during 2006-2008, and to cover as much as 252 000 ha. Latchininsky (2017) noted that the altitude of nesting areas of DMA has increased by 1600-1800 meter a.s.l in the 21st century. However, a similar altitude for nesting sites was documented as long as a hundred years ago (Syazov, 1912). At the same time, the boundaries of the known foci have shifted to the north Kokanova (2017). Fig. 6 compares the data from the model with the previously known nesting foci of DMA (Predtechensky et al., 1935) and confirms the spatial dynamics of the nesting areas of DMA. The optimal ranges may shrink or extend; and, what is of particular importance, the model reveals optimal nesting conditions in some areas of Central Asia where foci were never recorded before (e.g. a hot spot on the Afghanistan-Turkmenistan boundary).
The known nesting areas of DMA may reduce due to human activity. In Uzbekistan, the plowing of virgin soil between Jizak and Rostovtsevo railway stations led to two species of acridids (Dociostaurus kraussi Tarb., D. maroccanus Thnb.) completely vanishing from the area. On the other hand, hardening of soil due to overgrazing provides comfortable conditions for nesting (Yakhontov, 1964).
A previously known (Predtechensky et al., 1935) focus “Alexandrovsky” for DMA nesting located on the border between Kazakhstan and Kyrgyzstan may now be assumed to be non-functioning (Fig. 6 indicated with arrow). The territory of this focus is currently heavily cultivated, leaving apparently no intact landscapes and no intact hard soils for locust breeding and egg-survival. The model, however, provides evidence for possible DMA breeding on the border between Turkmenistan and Afghanistan (Fig. 6, indicated with dark circle). This spot possesses high suitability in terms of the key variables and with no signs of human activity. The area lies within the BSk zone by Köppen that is highly favorable for DMA egg development. It would be a desirable next step to study this area by ground methods in order to evaluate its status as a possible focus for nesting activity by Dociostaurus maroccanus.
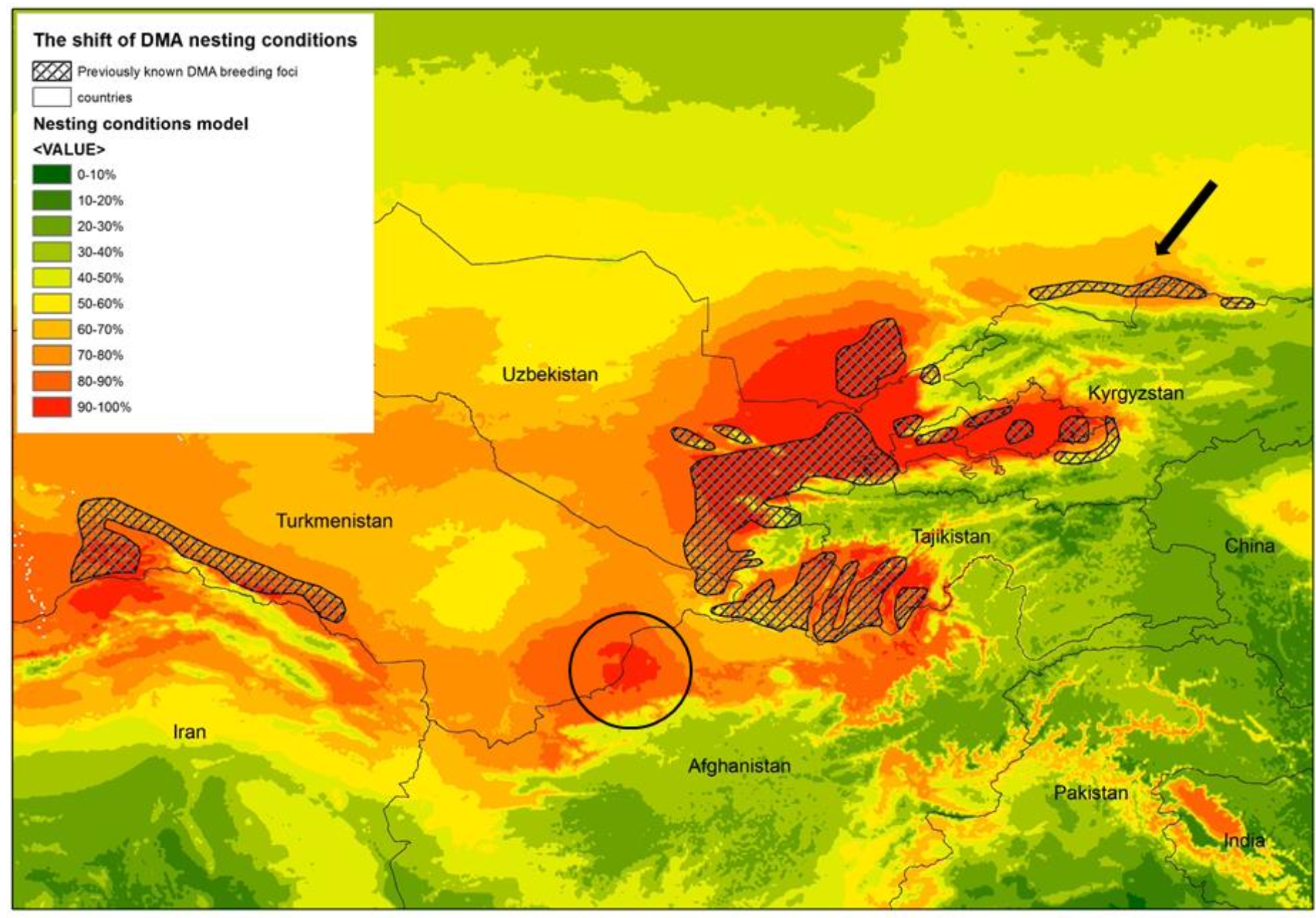
Conclusion
The model developed does not conflict with the known spatial distribution of DMA nesting foci. In terms of SDM, the model reveals good results. Moreover, the model has highlighted an area with suitable nesting conditions which has never been described before. This area (a hotspot at the boundary between Afghanistan and Turkmenistan) requires evaluation on the ground. The model also confirms significant trends in the spatial shift of nesting foci. Environmental factors, designated as “key variables” during the modeling procedure, fit well to known data regarding the species’ biology and physiology. More than half of the key variables (57%) are related to the winter and spring seasons, i.e. the most vulnerable stages of the locust’s life-cycle: embryogenesis and nymph development. The winter and spring variables, along with some of the autumn ones, describe the optimal conditions for the Moroccan locust’s nesting and embryogenesis.
40 variables are related to thermal parameters, influencing either the body temperature of nymphs and adults; or the thermal conditions for the survival of egg- pods throughout the cold seasons. 33 variables are concerned with humidity (evapotranspiration, precipitation, relative air humidity, and vapor pressure) during, mainly, the late winter and spring, when embryogenesis switches from diapause to normal development. The variables have a complicated interrelationship to each other and are basically related to hydrothermal conditions, the locust’s wellbeing and the persistence of vegetation. The multifaceted relationships between separate climatic variables were briefly described. It is virtually impossible to discuss each given variable separately, as their complicated interrelationships must be considered as a complex natural phenomenon, where every single part is dependent on the others. Validation of the model with ground data of 2018 regarding the distribution of egg- pods in Southern Kazakhstan demonstrated a good concordance between the model and the ground data. It should be noted, however, that modeling of the ecological niche remained extremely difficult and sometimes controversial due to several limitations(Aragón et al., 2012). It is impossible to encompass all of the complexity of biotic interactions. Moreover, insufficient information is available to incorporate adaptation or niche evolution and phenotypic plasticity, an extreme example of the latter being the change from the solitary phase to the gregarious phase that occurs in several locust species. The problem still remains and indeed increases regarding the incorporation of biotic variables into the model. Certain parameters, such as the condition of vegetation, derived from satellite information, appear to be quite easy to use within the models; whereas such factors as infraspecific or prey-predator relations are hard to formalize and to georeference for spatial modeling. Current knowledge is not sufficient for the incorporation of this information within distribution models (Aragón et al., 2012). Guisan & Thuiller (2005) described environmental predictors as falling into the following categories: (a) limiting factors (or regulators), defined as factors controlling species eco-physiology (e.g. temperature, water, soil composition); (b) disturbances, defined as all types of perturbations affecting environmental systems (natural or human- induced); and (c) resources, defined as all compounds that can be assimilated by organisms (e.g. energy and water). Of those categories, the limiting factors appear to be easier to incorporate for modeling with GIS. However, the last two categories require attention and it is necessary to understand all the possible ways in which they can be used within the modeling framework. First and most basically, one should ponder whether the model is used to investigate and understand the environmental correlates of a species’ distribution (explanation); or whether it is used to make inferences about the detail of their geographic distribution (prediction). If prediction is the focus, then it is important to know which component of the species’ geographic or environmental range is being estimated
(Araújo & Peterson, 2012). Of considerable importance is the response of an animal to unfavorably extreme conditions. Inasmuch as temperature varies widely among habitats, it is important to relate the tolerances of animals to conditions prevailing around them (Heatwole, 1983). Even when conditions are not life- threatening directly, they may be inimical to reproduction and thus might pose problems for the indefinite continuance of the species.
The advantages of the ENM procedure are clear. Ecological niche modeling provides results very closely related to ground and experimental data. The values of the key variables as obtained from ground research are similar if not identical to those obtained from the model. One the one hand, this justifies the ENM approach in general; and on the other, the model has the potential to attract the attention of the specialist to the influence of some previously underestimated variables. The procedure is cost-effective and the model itself provides good and extended information on the distribution and biology of the species being studied. However, in spite of the ENM approach being very promising and starting to be applied, it remains obvious that only a multidisciplinary approach incorporating the knowledge and effort of experts from many fields of science will be successful and will provide comprehensive results.
Acknowledgments
We thank Dr. V. E. Kambulin (Zhyembayev’s Institute of Plant Protection and Quarantine, Almaty) and Dr. V. I. Pankov (Republic Center for Phytosanitary and Prognosis, Pavlodar) for the providing of 2018 field data. Dr. T.N. Dujsebayeva (institute of Zoology, Almaty) has our gratitude for valuable comments she provided during the manuscript preparation. We are grateful to R. Sim (http://www.expert-english.com/) for correction of the English. This work was carried out under the auspices of the Republican budget program 008 “Applied Scientific Research in the Field of Space Activities”.
Conflicts of interest
Authors claim no conflict of interests with any person and/or organization.
References
Ahmad, M. F., & Rasul, G. (2008). Prediction of soil temperature by air temperature; a case study for Faisalabad. Pakistan Journal of Meteorology, 5(9), 19-27.
Allen, R. G., Pereira, L. S., Raes, D., & Smith, M. (1998). Crop evapotranspiration-Guidelines for Computing Crop Water Requirements-FAO Irrigation and drainage paper 56. Rome, Italy: FAO.
Aragón, P., Coca-Abia, M. M., Llorente, V., & Lobo, J. M. (2012). Estimation of climatic favourable areas for locust outbreaks in Spain: integrating species’ presence records and spatial information on outbreaks. Journal of Applied Entomology, 137(8), 610-623. https://doi.org/10.1111/jen.12022
Araújo, M. B., & Peterson, A. T. (2012). Uses and misuses of bioclimatic envelope modeling. Ecology, 93(7), 1527-1539.
Arias, G. A., Jiménez, V. J., & Pérez, R. A. (1997). Observaciones sobre el desarrollo embrionario y el avivamiento de Dociostaurus maroccanus (Thunb) en una finca de «La Serena» (Extremadura). Boletín de Sanidad V egetal Plagas, 23, 113-132.
Azhbenov, V. K., Baibussenov, K. S., Sarbaev, A. T., & Harizanova, V. B. (2015). Preventive approach of phytosanitary control of locust pests in Kazakhstan and adjacent areas. Paper presented at the International Conference on Agricultural, Ecological and Medical Sciences (AEMS-2015), Malaysia.
Barranco, P., Cabello, T., & Pascual, F. (1997). Cartografiado y hábitat de la langosta marroquí, Dociostaurus maroccanus (Thunberg, 1815) en la provincia de Almería. Boletin de la Asociacion Espanola de Entomologia, 21, 99-110.
Beard, J. T., & Gainer, J. L. (1969). Influence of solar radiation reflectance on water evaporation. Journal of Geophysical Research, 75(27), 5155- 5163.
Beaumont, L. J., Hughes, L., & Poulsen, M. (2005). Predicting species distributions: use of climatic parameters in BIOCLIM and its impact on predictions of species’ current and future distributions. Ecological Modelling, 186(2), 251- 270. https://doi.org/10.1016/j.ecolmodel.2005.01.030
Ben Halima, T., Gillon, Y., & Louveaux, A. (1985). Spécialisation trophique individuelle dans une population de Dociostaurus maroccanus (Orthopt.: Acrididae). Acta Oecologica Generalis, 6, 17-24.
Ben Halima, T., Gillon, Y., & Louveaux, A. (1984). Utilisation des ressources trophiques par Dociostaurus maroccanus (Thunberg, 1815) (Orth. Acrididae). Choix des espèces en fonction de leur valeur nutritive. Acta Oecologica Generalis, 5(4), 363-406.
Bey-Bienko, G. Y., & Mischenko, L. L. (1951). Acridids of the USSR and neighboring countries. AS USSR, 1, 378.
Bindi, M., & Miglietta, F. (1991). Estimating daily global radiation from air temperature and rainfall measurements. Climate Research, 1, 117-124. https://doi.org/10.3354/cr001117
Booth, T. H., Nix, H. A., Busby, J. R., & Hutchinson, M. F. (2013). bioclim: the first species distribution modelling package, its early applications and relevance to most currentMaxEntstudies. Diversity and Distributions, 20(1), 1-9. https://doi.org/10.1111/ddi.12144
Bounechada, M. (2007). Orthoptera research. Bioecological study and biological control tests on Ocneridia volxemi Bol. (Orthoptera, Pamphagidae) in the Sétif region. (Unpublished doctoral dissertation). Department of Biology, Faculty of Sciences.
Chen, J. L., & Li, G. S. (2014). Retraction statement: Estimation of monthly mean solar radiation from air temperature in combination with other routinely observed meteorological data in Yangtze River Basin in China. Meteorological Applications, 21(2), 459-459. https://doi.org/10.1002/met.1306
Daut, I., Yusoff, M. I., Ibrahim, S., Irwanto, M., & Nsurface, G. (2012). Relationship between the Solar Radiation and Surface Temperature in Perlis. Advanced Materials Research, 512-515, 143-147. https://doi.org/10.4028/www.scientific.net/amr.5 12-515.143
Dempster, J. P. (1963). The population dynamics of grasshoppers and locusts. Biological Reviews, 38(4), 490-529. https://doi.org/10.1111/j.1469- 185x.1963.tb00791.x
Dujsebayeva, T. N., & Malakhov, D. V. (2017). The model of Ranodon sibiricus ecological niche: GIS and Remote Sensing Approach. Russian Journal of Herpetology, 24(3), 171-192.
Foster, J. A., & Robertson, R. M. (1992). Temperature dependency of wing-beat frequency in intact and deafferented locusts. Journal of Experimental Biology, 162(1), 295-312.
Franklin, J. (2010). Mapping species distributions: Spatial inference and prediction. Cambridge University Press: Cambridge.
Graham, C. H., & Hijmans, R. J. (2006). A comparison of methods for mapping species ranges and species richness. Global Ecology and Biogeography,15(6),578-587. https://doi.org/10.1111/j.1466- 8238.2006.00257.x
Guisan, A., & Thuiller, W. (2005). Predicting species distribution: offering more than simple habitat models. Ecology Letters, 8(9), 993-1009.
Heatwole, H. (1983). Physiological responses of animals to moisture and temperature. In F.B. Golley (ed.), Tropical Rain Forest Ecosystems. Amsterdam: Elsevier.
Hijmans, R. J., Cameron, S. E., Parra, J. L., Jones, P. G., & Jarvis, A. (2005). Very high resolution interpolated climate surfaces for global land areas. International Journal of Climatology, 25(15), 1965-1978. https://doi.org/10.1002/joc.1276
Houlder, D., Hutchinson, M., Nix, H., & McMahon, J. (2001). ANUCLIM. Canberra: Centre for Resource and Environmental Studies.
Hutchinson, G. E. (1957). Concluding Remarks. Cold Spring Harbor Symposia on Quantitative Biology, 22(0), 415-427. https://doi.org/10.1101/sqb.1957.022.01.039
Islam, K. I., Khan, A., & Islam, T. (2015). Correlation between atmospheric temperature and soil temperature: a case study for Dhaka, Bangladesh. Atmospheric Climate Sciences, 5(03), 200.
Ituen, E. E., Esen, N. U., Nwokolo, S. C., & Udo, E. G. (2012). Prediction of global solar radiation using relative humidity, maximum temperature and sunshine hours in Uyo, in the Niger Delta Region, Nigeria. Advances in Applied Science Research, 3(4), 1923-1937.
Kokanova, E. O. (2017). Natural foci of the Moroccan locust (Dociostaurus maroccanus, Orthoptera, Acrididae) in Turkmenistan and their current state. Entomological Review, 97(5), 584-593. https://doi.org/10.1134/s0013873817050049
Kottek, M., Grieser, J., Beck, C., Rudolf, B., & Rubel, F. (2006). World Map of the Köppen-Geiger climate classification updated. Meteorologische Zeitschrift, 15(3), 259-263. https://doi.org/10.1127/0941-2948/2006/0130
Latchininsky, A. V. (2017). Climate changes and locusts: what to expect? Uch. Zap. RGGU, 46, 134-143.
Latchininsky, A. V., Sergeev, M. G., Childebaev, M. K., Chernijakhovskij, M. E., Lockwood, J. A., Kambulin, V. E., & Gapparov, F. A. (2002). The grasshoppers of Kazakhstan, Middle Asia and adjacent territories. Laramie: International Association of Applied acridology and University of Wyoming.
Lawrence, M. G. (2005). The Relationship between Relative Humidity and the Dewpoint Temperature in Moist Air: A Simple Conversion and Applications. Bulletin of the American Meteorological Society, 86(2), 225-234. https://doi.org/10.1175/bams-86-2-225
Malakhov, D. V., & Chirikova, M. A. (2018). Species Distribution Model of Varanus griseus caspius (Eichwald, 1831) in Central Asia: an Insight to the Species’ Biology. Russian Journal of Herpetology, 25(3), 195.206. https://doi.org/10.30906/1026-2296-2019-25-3- 195-206
Malakhov, D. V., Tsychueva, N. Y., & Vitkovskaya, I. S. (2017). Modelling the ecological niche of wheat septoriosis using remote sensing data. Sovremennye problemy distantsionnogo zondirovaniya Zemli iz kosmosa, 14(1), 113-124. https://doi.org/10.21046/2070-7401-2017-14-1- 113-124
Malakhov, D. V., Tsychuyeva, N. Y., & Kambulin, V. E. (2018). Ecological modeling of Locusta migratoria L. breeding conditions in South- Eastern Kazakhstan. Russian Journal of
Ecosystem Ecology, 3(1).
https://doi.org/10.21685/2500-0578-2018-1-5 Miller, G. A., Clissold, F. J., Mayntz, D., & Simpson, S. J. (2009). Speed over efficiency: locusts select body temperatures that favour growth rate over efficient nutrient utilization. Proceedings. Biological sciences, 276(1673), 3581-3589.
Monar, A., Shiris, M., & Lachininsky, A. (2009). The Acridid situation and the struggle with locusts in Caucasus and Central Asia. Rome, Italy: Food and Agriculture Organization.
Peterson, A. T., & Soberón, J. (2012). Species Distribution Modeling and Ecological Niche Modeling: Getting the Concepts Right. Natureza & Conservação, 10(2), 102-107. https://doi.org/10.4322/natcon.2012.019
Popova, E. N., & Popov, I. O. (2009). Harmful acridids of Southern Russia and climatic factors, regulating their breeding and distribution. Problems of ecological monitoring and modeling of ecosystems. Moscow, 22, 124-146.
Prange, H. D. (1990). Temperature regulation by respiratory evaporation in grasshoppers. Journal of Experimental Biology, 154, 463-474.
Predtechensky, S. A., Zhdanov, S. P., & Popova, A. A. (1935). Harmful acridids in USSR. VASKHNIL. Proceedings of Plant Protection, 18, 167.
Quesada-Moraga, E., & Santiago-Álvarez, C. (2001). Assessment of sexual maturation in the Moroccan locust Dociostaurus maroccanus (Thunberg). Journal of Orthoptera Research, 10(1), 1-8. https://doi.org/10.1665/1082- 6467(2001)010[0001:aosmit]2.0.co;2
Rouse, J. W., Haas, R. H., Schell, J. A., & Deering, D. W. (1974). Monitoring vegetation systems in the Great Plains with ERTS. In Third ERTS Symposium (pp. 309-317): NASA SP-351 I.
Santiago‐Alvarez, C., Quesada‐Moraga, E., & Hernández‐Crespo, P. (2003). Diapause termination and post‐diapause development in the Mediterranean locust Dociostaurus maroccanus (Orth., Acrididae) under field conditions. Journal of Applied Entomology, 127(6), 369-373.
Soberón, J., & Peterson, T. (2005). Interpretation of models of fundamental ecological niches and species’ distributional areas. Biodiversity informatics, 2, 1-10.
Song, H. (2011). Density-Dependent Phase Polyphenism in Nonmodel Locusts: A Minireview. Psyche: A Journal of Entomology, 2011, 1-16. https://doi.org/10.1155/2011/741769
Swartman, R. K., & Ogunlade, O. (1967). A statistical relationship between solar radiation,’ sunshine and relative humidity in the tropics. Atmosphere, 5(2), 25-34. https://doi.org/10.1080/00046973.1967.9676534
Syazov, M. (1912). The struggle with locusts in Turkestan, 1901-1911. In (pp. 33). Tashkent.
Tronin, A. A. (2007). Temporal series of satellite data and the prognosis of locust’s outbreaks in Western Siberia and Kazakhstan. Curr. Probl. Remote Sensing of the Earth from Space, 4, 390- 394.
Tsyplenkov, E. P. (1970). Harmful Acridids in USSR. Leningrad.
UNEP (United Nations Environment Programme). (1997). World atlas of desertification (2 ed.). London: UNEP.
Uvarov, B. P. (1955). The aridity factor in the ecology of Locusts and Grasshoppers of the Old World.
Winslow, J. C., Hunt, E. R., & Piper, S. C. (2001). A
globally applicable model of daily solar irradiance estimated from air temperature and precipitation data. Ecological Modelling, 143(3), 227-243. https://doi.org/10.1016/s0304- 3800(01)00341-6
Yakhontov, V. V. (1964). Physiology of the Insects (in Russian). Moscow.