Authors: Nazerke Bizhanova, Moriz Steiner, Nurkuisa Rametov, Alexey Grachev, Yuri Grachev, Maxim Bespalov, Tungyshbek Zhaparkulov, Saltore Saparbayev, Amanbol Sailaukhanuly, Sergey Bespalov, Aibol Bolatuly, Kuandyk Saparov and Shahrul Anuar Mohd Sah
DOI: https://doi.org/10.3390/su14159491
Abstract:
The Turkestan lynx (Lynx lynx isabellina Blyth, 1847) is a rare and understudied subspecies of the Eurasian lynx occupying the mountains of Central and South Asia. This elusive felid’s northwestern range includes the Tien Shan and Zhetisu Alatau mountains in the border region of Kazakhstan, China, Kyrgyzstan, and Uzbekistan. As the first step to conserve this vulnerable carnivore, we have conducted the first full-scale research from 2013 until 2022 on its distribution in this region. Using 132 environmental predictors of 359 lynx sightings, we have created species habitat distribution models across the lynx’s northwestern range using machine learning approaches (Maximum Entropy—MaxEnt).
Additionally, we created species distribution forecasts based on seven bio-climatic environmental predictors with each three different future global climate model scenarios. To validate these forecasts, we have calculated the changes in the lynx distribution range for the year 2100, making the first species distribution forecast for the Turkestan lynx in the area. Additionally, it provides insight into the possible effects of global climate change on this lynx population. Based on these distribution models, the lynx population in the Northern and Western Tien Shan and Zhetisu Alatau plays a significant role in maintaining the stability of the whole subspecies in its northwestern and global range, while the distribution forecast shows that most lynx distribution ranges will reduce in all future climate scenarios, and we might face the Turkestan lynx’s significant distribution range decline under the ongoing and advancing climate change conditions. For a future (year 2100) warming scenario of 3 deg. C (GCM IPSL), we observe a decrease of 35% in Kazakhstan, 40% in Kyrgyzstan, and 30% in China as the three countries with the highest current predicted distribution range. For a milder temperature increase of 1.5–2 deg. C. (GCM MRI), we observe an increase of 17% Kazakhstan, decrease of 10% in Kyrgyzstan, and 57% in China. For a cooling scenario of approx. 1–1.5 deg. C (GCM MIROC), we observe a decrease of 14% Kazakhstan, increase of 11% in Kyrgyzstan, and a decrease of 13% in China. These modeled declines indicate the necessity to create new and expand the existing protected areas and establish ecological corridors between the countries in Central and South Asia.
You can download PDF-version of article by clicking here!
You can see article page on the Sustainability journal by clicking here!
You can see article page on the Researchgate by clicking here!
Authors:
Nazerke Bizhanova (1,2,3,*), Moriz Steiner (4,5,6), Nurkuisa Rametov (7), Alexey Grachev (2,3,*), Yuri Grachev (2), Maxim Bespalov (2), Tungyshbek Zhaparkulov (8), Saltore Saparbayev (2), Amanbol Sailaukhanuly (9), Sergey Bespalov (2), Aibol Bolatuly (10), Kuandyk Saparov (1) and Shahrul Anuar Mohd Sah (11)
1 – Department of Biodiversity and Bioresources, Al-Farabi Kazakh National University, Almaty 050040, Kazakhstan; kuandyk.saparov@kaznu.kz
2 – Laboratory of Theriology, Institute of Zoology, Almaty 050060, Kazakhstan; yuriy.grachev@zool.kz (Y.G.); maksim.bespalov@zool.kz (M.B.); saltore.saparbaev@zool.kz (S.S.); sergey.bespalov@zool.kz (S.B.)
3 – Wildlife Without Borders Public Fund, Almaty 050063, Kazakhstan
4 – Department of Animal Science, Wageningen University and Research, 6708 PB Wageningen, The Netherlands; moriz.steiner@wur.nl
5 – IUCN Small Mammal Specialist Group (SMSG), IUCN, Rue Mauverney 28, 1196 Gland, Switzerland
6 – IUCN Species Survival Commission (SSC), IUCN, Rue Mauverney 28, 1196 Gland, Switzerland
7 – Department of Geospatial Engineering, Satpaev Kazakh National Research Technical University, Almaty 050000, Kazakhstan; n.rametov@stud.satbayev.university
8 – Department of Research and Mountain Agrobiocenosis, Ile-Alatau National Park, Almaty 050067, Kazakhstan; nauka.ile-alatau@mail.ru
9 – Almaty State Nature Reserve, Almaty 041600, Kazakhstan; amanbol.saylaukhanuly@mail.ru
10 – Kolsai Kolderi National Park, Saty 041422, Kazakhstan; myrzahanov_aibol@mail.ru
11 – School of Biological Sciences, Universiti Sains Malaysia, Gelugor 11800, Malaysia; sanuar@usm.my
* – Correspondence: nazerke.bizhanova@zool.kz (N.B.); alexey.grachev@zool.kz (A.G.)
Keywords: Eurasian lynx; Turkestan lynx; Tien Shan; Dzhungarian (Zhetisu) Alatau; species dis- tribution models; species distribution forecast; MaxEnt; environmental predictors; protected areas; conservation
Introduction
The development of industry and agriculture, as well as a significant increase in the world’s population, have led to an increase in anthropogenic pressure on natural ecosystems, fauna and flora [1–3]. In particular, human activities have precipitated habitat loss and fragmentation, followed by isolation, loss of genetic diversity and biodiversity, decline in population sizes, and extinction of wild animals [4–8]. Among vertebrates, a number of large carnivorous mammals are threatened with extinction due to their lower population density and reproductive rates. Moreover, some of the main threats to carnivores are related to people’s prevailing negative attitude towards these animals, which may result in their direct or indirect extermination [9].
The Eurasian lynx (Lynx lynx L., 1758) is a felid species especially affected by human activities [10]. For instance, as a species that was once widespread throughout Europe, it became extinct in the region in the 19th century due to habitat loss and persecution [11,12]. The lynx is still considered a rare or endangered species, even after its re-introduction in a number of countries [13,14]. The case of lynx disappearance in Europe is a good example that indicates the need for measures to study and preserve this species and its subspecies in other habitats, taking into account the evolving industry and intensive developing of natural areas.
The Turkestan lynx, also known as Himalayan lynx, Central Asian lynx (Lynx lynx isabellina, also referred to as Lynx lynx isabellinus Blyth, 1847) is an elusive subspecies of the Eurasian lynx that occupies the mountains of Central and South Asia. Being rare and understudied throughout all 11 countries of its habitat, the lynx is listed in Appendix II of the International CITES Convention. In Central Asia, this carnivore is listed in the Red Data Book of Kazakhstan as “a rare subspecies, the range and number of which are decreasing” (III category) [15]; Kyrgyzstan as a “subspecies close to a vulnerable position” (NT, VI category), [16]; Uzbekistan as a “vulnerable declining subspecies” (II category—VU: D) [17]; Turkmenistan as “an extinct or endangered species or subspecies” (Category I) [18]; Tajikistan as an “endangered subspecies” (EN) [19]; China as an “endangered subspecies” (EN A1cd) [20]; and in Afghanistan, the lynx is considered “vulnerable” [21,22]. In South Asia, the lynx is common in the northern part of Pakistan, and it is considered to be of “Least Concern” (LC) in the region [23]. However, the current status and distribution in Pakistan is unknown [24], which confirms the need to update the Red List in Pakistan [23,25]. In India, the lynx is on the verge of extinction [26,27], and is listed in Plan I—protected by the National Indian Wildlife Protection Act since 1972. The lynx is listed in the Red Data Book of Nepal as a “vulnerable subspecies” (Category Vulnerable B1a; D2; protected under the National NPWC Act 2029) [28]. In Bhutan, there is no information available to determine lynx status (Data Deficiency).
The study is complicated by the inaccessibility of lynx habitats, as well as its secretive lifestyle. As it is exceedingly elusive, this form of the lynx is poorly studied, especially in the northwest of its global range, where it inhabits the mountains of Northern and Western Tien Shan and Dzhungarian (Zhetisu) Alatau [29]. The mentioned mountains are located in the border region of Kazakhstan, China, Kyrgyzstan, and Uzbekistan.
Most of the early studies conducted on a local scale in Kazakhstan in the 20th century were mainly related to the general distribution of the species, indicating the lynx presence in a particular mountain range [30–32], or contain fragmentary data on lynx sightings obtained while studying other mammalian species [33–41]. The situation is similar in neighboring Kyrgyzstan [16,40,42–53] and Uzbekistan [54–65]. More extensive studies in China by Ablimit et al. [66] indicate that, in the northwestern region, which includes the Xinjiang Autonomous Region, suitable lynx habitats in this region have decreased by 50% since the 1950s. At the same time, data on lynx in China are still insufficient [67], and detailed studies on lynx distribution are needed in the country [68].
In the northwest of its distribution, one of the key lynx habitats in Central Asia, the Turkestan lynx populations are understudied and heavily affected by habitat degradation and fragmentation, prey base depletion, poaching, and conflict with livestock breeders (fur trade and retaliatory killings due to livestock depredation) [69]. With the intensive regional development, the habitats may reduce in size and quality, thus increasing the extinction rate of lynx populations and subpopulations in these mountains [70]. As the first step in preserving this felid, we will predict the subspecies’ distribution, as it is an important component of populations and species conservation planning, and a variety of modeling techniques have been developed for this purpose [71].
Our study goals are twofold: (i) relying on 132 relevant environmental characteristics and our recently obtained more accurate lynx occurrence records, assess the habitat suitabil- ity for the lynx populations and create the first subspecies distribution model (SDM) in its northwest range; (ii) based on seven bio-climatic environmental characteristics, modelling a forecast on the lynx habitat distribution—subspecies distribution forecast (SDF) for the year 2100, thus evaluating the effect of climate change on the lynx’s distribution range. For this purpose, we will apply the above-mentioned models, also used in different modifications by other researchers to study the Eurasian lynx distribution in the world [72–75].
2. Materials and Methods
2.1. Study Area
We collected the data from the Northern Tien Shan, Western Tien Shan, and the Zhetisu Alatau mountains (Figure 1). We conducted our research within Kazakhstan, located in the most northwestern edge of the Turkestan lynx’s geographical range, and analyzed the survey and literature data from China, Kyrgyzstan, and Uzbekistan.
- In the Northern Tien Shan, our study area covered the mountain ranges—Ile Alatau (N 42◦58′–43◦47′, E 74◦53′–78◦54′), Kungei Alatau (N 42◦47′–43◦01′, E 77◦18′–78◦59′), and Terskei Alatau (N 42◦28′–42◦46′, E 79◦13′–80◦07′), located in the border area of Kazakhstan and Kyrgyzstan, and Uzynkara (Ketmen) (N 43◦02′–43◦25′, E 79◦17′– 80◦40′), located in the border area of Kazakhstan and China. In the Xinjiang part of Northern Tien Shan, we considered the felid occurrences in the Tomur (N 41◦54′, E 80◦18′), Kalajun–Kuerdening (N 42◦59′–43◦05′, E 82◦22′–82◦59′), Bayinbuluke (N 42◦47′, E 84◦09′) and Bogda (N 43◦51′, E 88◦09′) areas. We also studied Kyrgyz Alatau (N 42◦38′–42◦40′, E 73◦14′–74◦35′), which is located between Kazakhstan and Kyrgyzstan, and is an interjacent ridge between Northern and Western Tien Shan.
- In the Western Tien Shan, we checked the lynx occurrences in the ranges of Karatau (N 42◦53′, E 69◦58′), located in Kazakhstan; Karzhantau (N 41◦50′–42◦03′, E 69◦50′– 69◦58′), Talas Alatau (N 42◦19′–42◦25′, E 70◦22′–71◦51′) and Ugam (N 41◦49′–42◦04′, E 70◦16′–70◦25′), located in the border region of Kazakhstan and Uzbekistan; Pskem (N 41◦45′–42◦02′, E 70◦22′–70◦41′) and Chatkal (N 41◦10′–41◦23′, E 69◦49′–70◦29′), located in the border between Uzbekstan and Kyrgyzstan.
- In the Zhetisu Alatau, located in the border region of Kazakhstan and China, we surveyed the ridges of Sholak (N 43◦56′, E 77◦56′), Degeres (N 44◦00′, E 78◦10′), Matai (N 44◦10′, E 78◦26′), Altynemel (N 44◦13′, E 78◦34′), Koyandytau (N 44◦21′, E 78◦49′), Toksanbay (N 44◦43′, E 79◦04′), Baskantau (N 44◦04′, E 80◦26′), Karatau (N 44◦57′, E 79◦38′), Bedzhintau (N 45◦02′, E 79◦58′), and Tyshkantau (N 44◦37′, E 80◦08′).
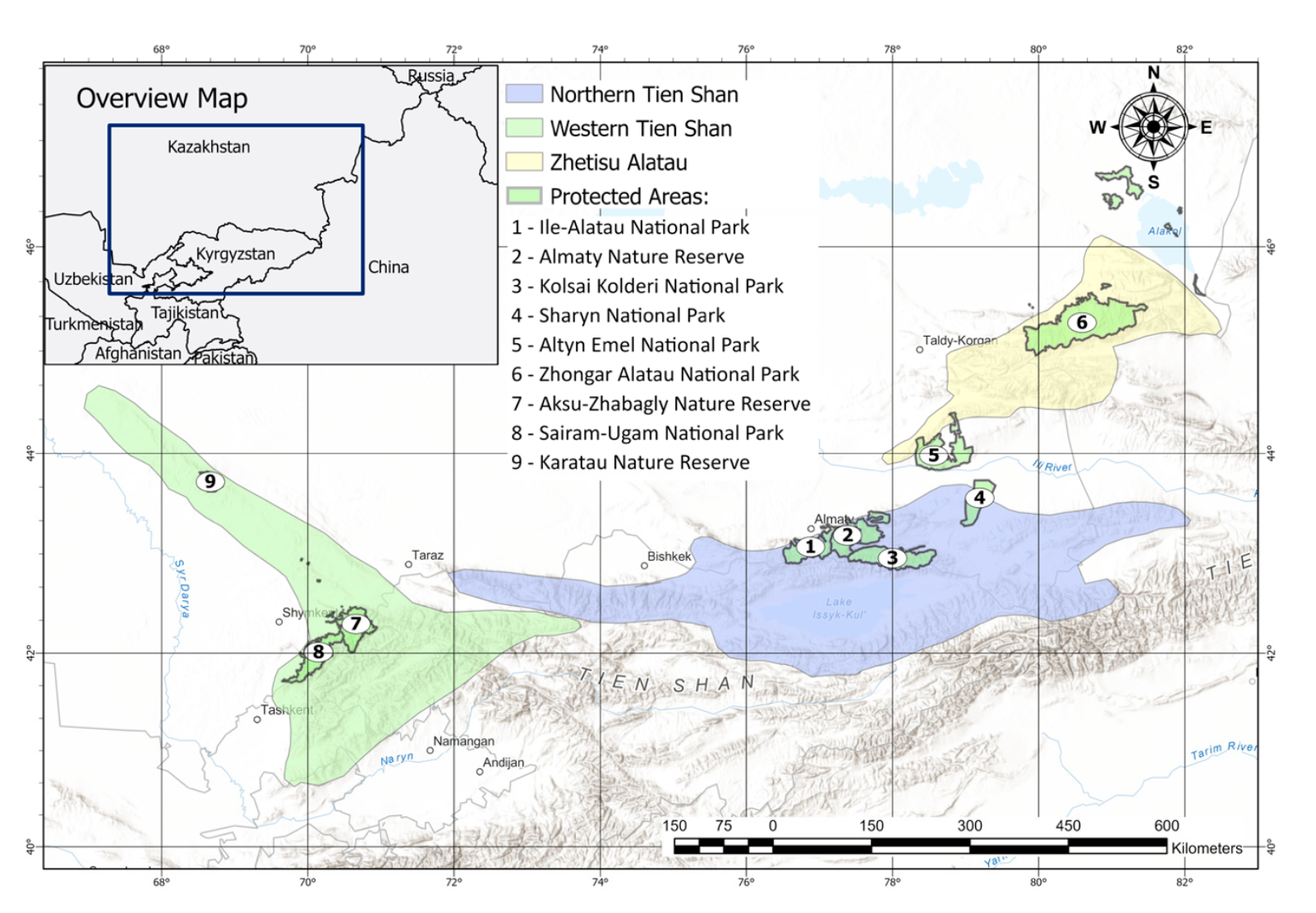
The Tien Shan ridges have a latitudinal or nearly latitudinal strike [76]. The climate in The Tien Shan ridges have a latitudinal or nearly latitudinal strike [76]. The climate the mountains is continental; nevertheless, the complexity and vegetation of the relief cause in the mountains is continental; nevertheless, the complexity and vegetation of the relief contrasts in temperature and the degree of moisture [77,78]. Thus, the average annual air cause contrasts in temperature and the degree of moisture [77,78]. Thus, the average an- temperatures in Tien Shan decrease to 6.5 ◦C below zero [79]; at the same time, the southern nual air temperatures in Tien Shan decrease to 6.5 °C below zero [79]; at the same time, slopes of the ridges can be 5–10 ◦C warmer than the northern ones [80].
the southern slopes of the ridges can be 5–10 °C warmer than the northern ones [80].
Most of the plant species are found in the mid-altitude mountain forest belt. Above Most of the plant species are found in the mid-altitude mountain forest belt. Above 2000–2200 m, deciduous forests give way to spruce on mountain–forest dark-colored soils 2000–2200 m, deciduous forests give way to spruce on mountain–forest dark-colored soils with a high (up to 10%) humus content [81,82]. The important component of the spruce with a high (up to 10%) humus content [81,82]. The important component of the spruce forests on the northern slope of the Northern Tien Shan ridges, particularly for the lynx forests on the northern slope of the Northern Tien Shan ridges, particularly for the lynx distribution, is the endemic species, the Schrenk’s spruce (Picea shrenkiana) [83,84]; in the distribution, is the endemic species, the Schrenk’s spruce (Picea shrenkiana) [83,84]; in the Western Tien Shan—the juniper shrubs (Juniperus pseudosabina, J. sibirica); and in the Zhetisu Western Tien Shan—the juniper shrubs (Juniperus pseudosabina, J. sibirica); and in the Zhe-Alatau—the Schrenk’s spruce mixed with the Siberian fir (Abies sibirica) [30].
tisu Alatau—the Schrenk’s spruce mixed with the Siberian fir (Abies sibirica) [30].
We collected the majority of occurrence data in specially protected natural areas (PAs): Ile-Alatau State National Natural Park (SNNP), Almaty State Nature Reserve (SNR), Kolsai Kolderi SNNP, Sharyn SNNP, Altyn Emel SNNP, Zhongar Alatau SNNP, Aksu-Zhabagly SNR, Sairam-Ugam SNNP, and Karatau SNR, and in the adjacent territories. The (SNR), Kolsai Kolderi SNNP, Sharyn SNNP, Altyn Emel SNNP, Zhongar Alatau SNNP, lynx populations in the PAs were comparably more stable, with a lower level of human disturbance and activities noted. General environmental conditions given in average for lynx locations within our study area are presented in Appendix A (Table A1).
2.2. Occurrence Data
2.2.1. Records Search and Analysis
We searched extensively for observational records within our study area. The literature data used in this study were collected mainly from zoological and ecological reports of the Institute of Zoology of Kazakhstan and Specially Protected Natural Areas (PAs), zoological articles, books, conference materials, methodological manuals, etc. In order to determine the modern lynx distribution, in the Northern Tien Shan, we frequently used the records in the diaries of the inspectors (rangers) of protected areas. In the Xinjiang part of Tien Shan, we mainly relied on the data given in the papers and reports of Ablimit et al. [42], the Leading Group for Application of WNH of Xinjiang Uygur Autonomous Region [87], and Tancheng Dong et al. [88], and we also examined the locations mentioned in craniological notes of lynx skulls by A. Regel (collected in 1880; Skulls No. 1164, 1284, 1287, 1325; Zoological Institute of Russian Academy of Science, St. Petersburg, Russia). One location presented in the gbif.org database was examined as well (accessed on 16 April 2022). In the Western Tien Shan, we often relied on the occurrence data by Bykova et al. [67] and Shakula et al. [89] and the oral communication of S.V. Baskakova. In the Zhetisu Alatau, one location given by V.A. Zhiryakov and B.R. Baidavletov [32] was considered for the lynx occurrence data. The reliability of all regions of lynx encounters, provided in scientific papers and reports, were reviewed and verified. Oral communications were taken from experienced researchers in the area.
2.2.2. Field Data Collection
During research from 2013 until 2022, we relied on traditional methods of field mam- malogical research [90,91], which included visual observations and identification of various lynx and their prey traces (paw and hoof prints, prey remains, feces, scratches on trees and rocks, etc.) with their registration on the GPS. We typically collected the data on field trips during winter, as it was more attainable to observe tracks on snow.
Within the Kazakh part of our study area, we completed horse-walking routes with a total length of 1426 km, with 586 km in the Ile Alatau, 185 km in the Kungei Alatau, 74 km in the Terskei Alatau, 32 km in the Uzynkara, and 54 km in the Kyrgyz Alatau ridges of Northern Tien Shan; 108 km in the Talas Alatau and 47 km in the Ugam ridge of Western Tien Shan; and 340 km in the Zhetisu Alatau.
2.2.3. Camera Trapping
Research with the use of camera traps was mainly carried out in areas where the level of anthropogenic pressure was reduced. The camera traps (models Bushnell, Reconyx, Seelock, ScoutGuard, BolyGuard, Browning) were installed in 143 locations from 2013 to 2022 in the most potential lynx habitats (Figure 2), which we selected taking into account our own and survey data, as well as the presence of its prey. For the calculation of lynx and its prey abundance in the study area, we used the formula below (see the results in the Section 4.5).
The average abundance was calculated using this formula:
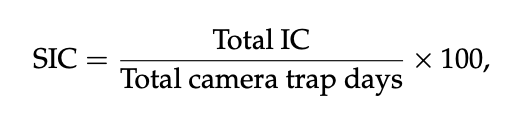
where IC means independent captures, SIC means IC per season (IC per 100 trap days), and camera trap days represents the number of applied camera traps multiplied by the number of days they were installed for.
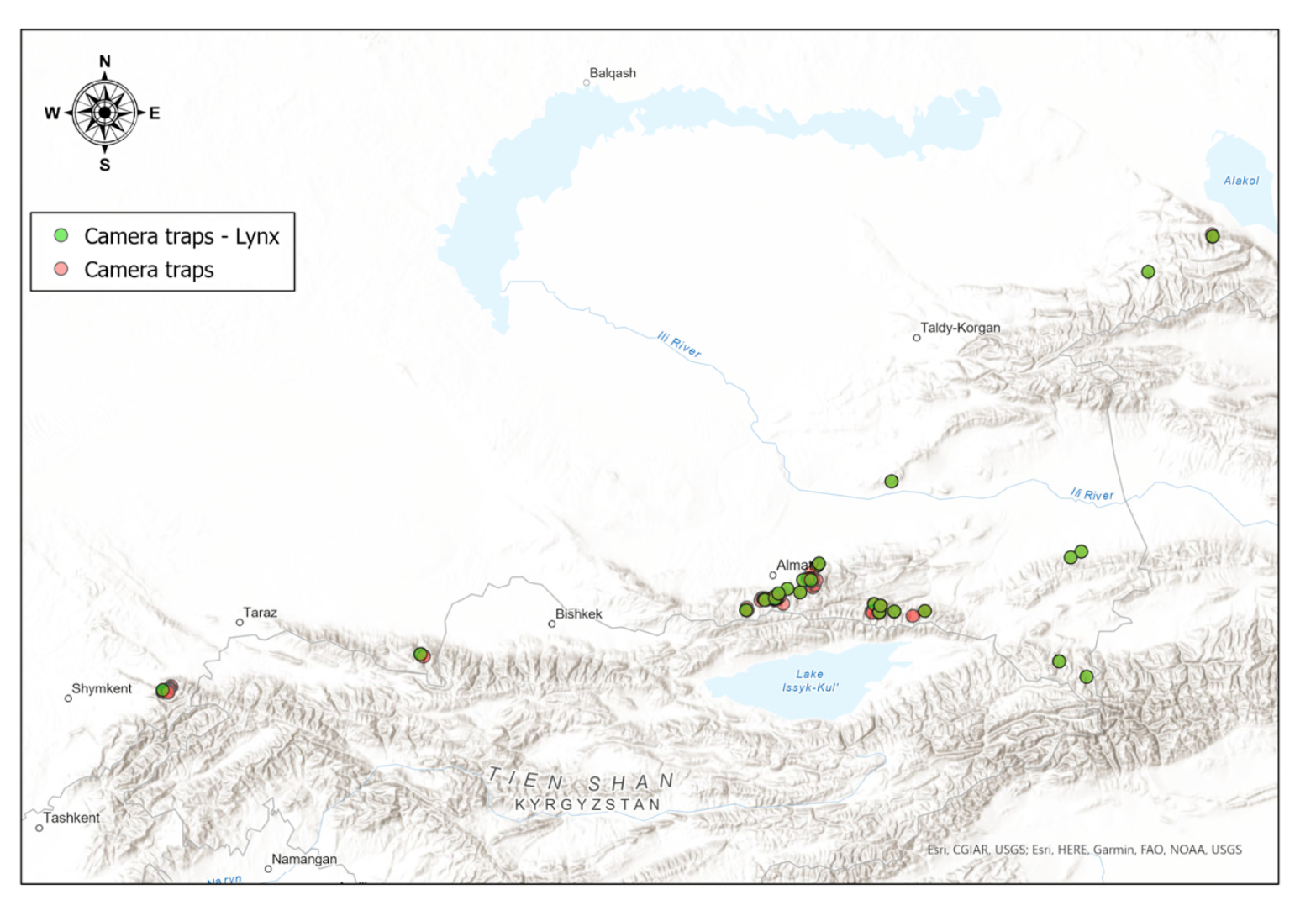
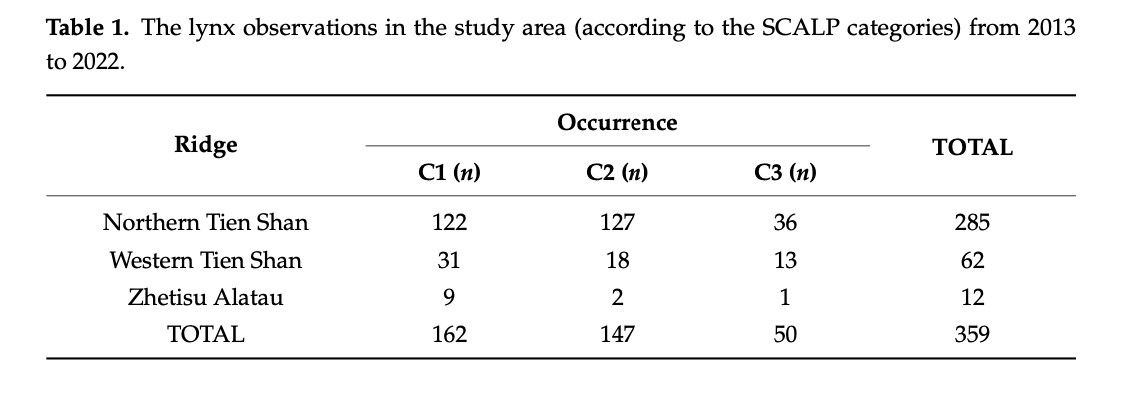
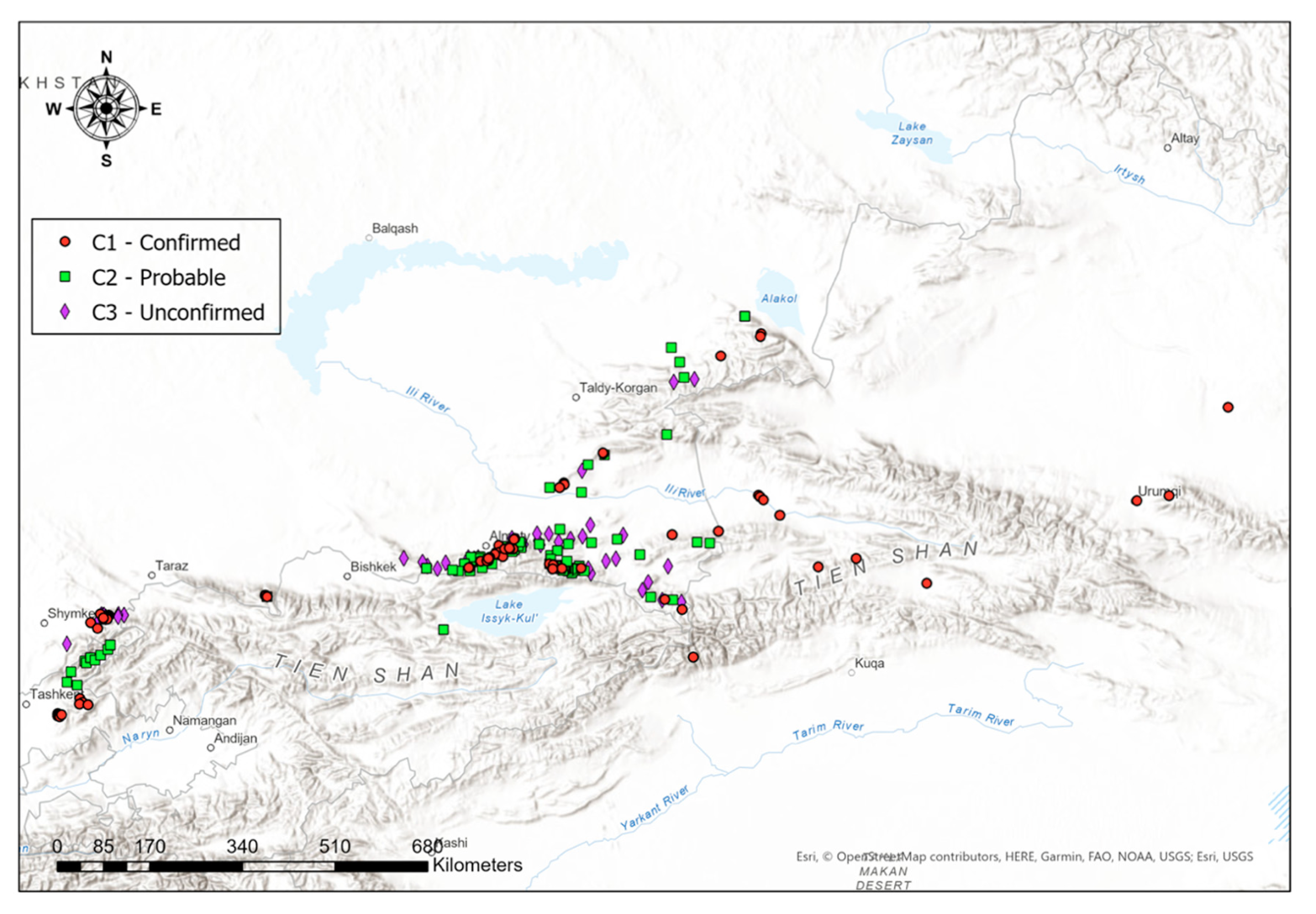
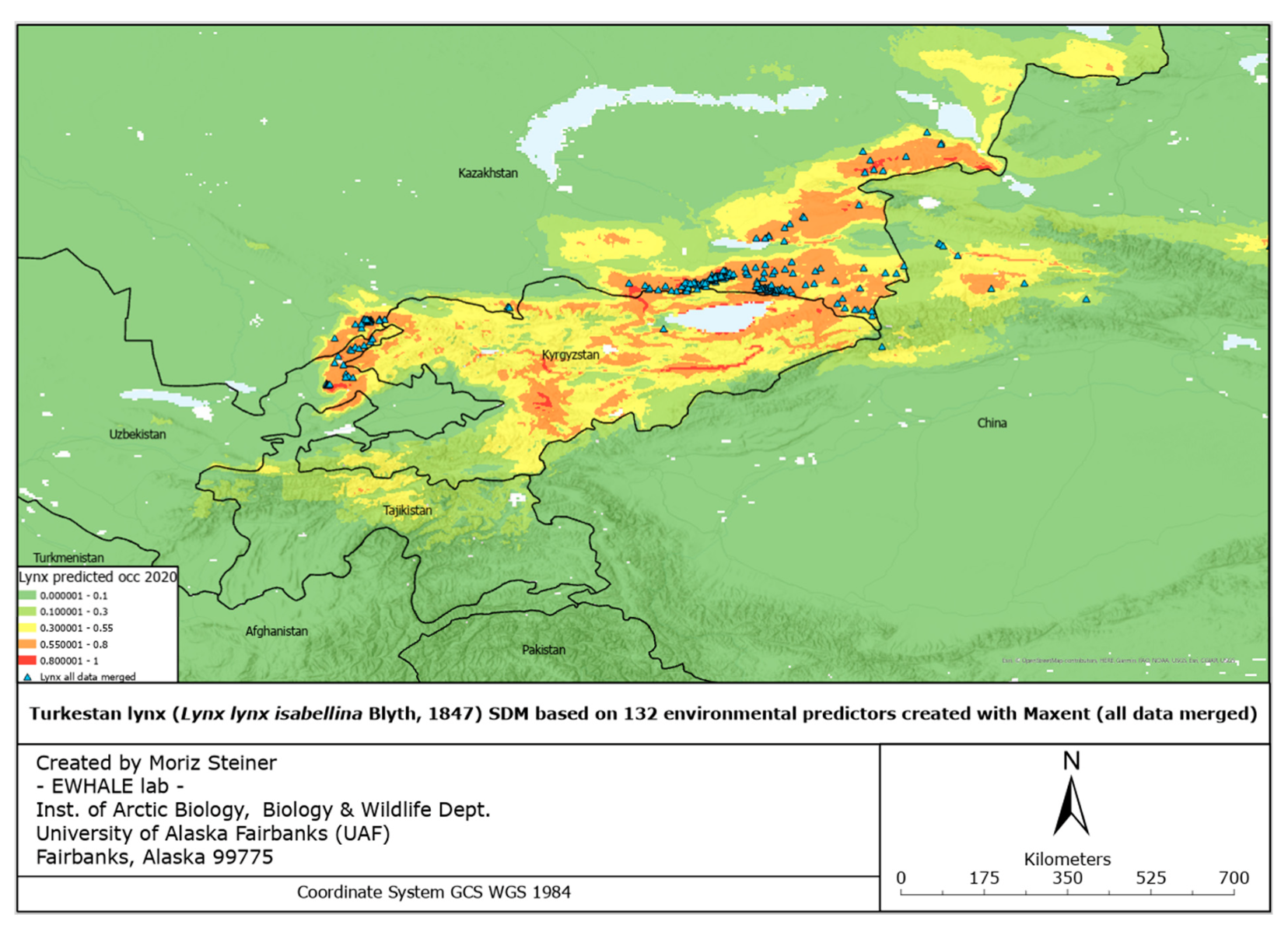
2.3 Environmental Predictors
2.3.1. Current Environmental Predictors
In this study, we created species distribution models (SDMs) based on 132 environmental predictors. These 132 environmental predictors aim to best describe the surrounding mental predictors. These 132 environmental predictors aim to best describe the surround- environmental and habitat conditions of the studied subspecies (Turkestan lynx (Lynx ing environmental and habitat conditions of the studied subspecies (Turkestan lynx (Lynx lynx isabellina)) (see [94]). These are the building blocks of the SDMs, apart from the col- lynx isabellina)) (see [94]). These are the building blocks of the SDMs, apart from the col- lected occurrence data [95]. These 132 predictors have been retrieved by MS from Steiner lected occurrence data [95]. These 132 predictors have been retrieved by MS from Steiner and Huettmann (in-press) [8]. They describe, e.g., the temperature, precipitation, rela- and Huettmann (in-press) [8]. They describe, e.g., the temperature, precipitation, relative tive humidity, soil conditions, snowfall, proximity to streets, cities and rivers, wildfires, humidity, soil conditions, snowfall, proximity to streets, cities and rivers, wildfires, vegetation cover, climate classes, prey densities, etc., on a global scale. All details and citation cover, climate classes, prey densities, etc., on a global scale. All details and sources sources of the 132 predictors can be found in Appendix A, Table A1 (Reproduced from of the 132 predictors can be found in Appendix A, Table A1 (Reproduced from Steiner Steiner and Huettmann (in-press)) [8]. Each environmental predictor has an accuracy of and Hu2ettmann (in-press)) [8]. Each environmental predictor has an accuracy of 2.5 km2 degrees (CRS: EPSG:4326—WGS 84). This is believed to be the most complete set of environmental (CRS: EPSG:4326—WGS 84). This is believed to be the most complete set of environmental predictors publicly available to this date.
2.3.2. Projected (Future) Climatic Predictors
We created species distribution forecasts (SDFs) based on seven environmental predictors. These SDSf aim to predict the distribution of Turkestan lynx for the year 2100 using bio-climatic environmental predictors only.
These predictors follow the same technical details (pixel size, accuracy) as the ones used for the SDMs above. The reason for only using using 7 predictors for these SDFs compared to the 132 predictors for the SDMs is that significantly less data are available for the year 2100 than 2000–2020. Since 2100 is in the relatively far future, for the models here we used three different scenarios. These three scenarios aim to cover three different climate directions that are likely to be approached by 2100. The three scenarios utilized in this study are Global Climate Models (GCMs) that have been downloaded from www.WorldClim.org (accessed on 1 June 2022) (MIROC6, MRI-ESM2-0, and IPSL-CM6A-LR). Similarly, as described by Steiner and Huettmann (in-press—Chapter 7) [8], the MIROC6 may be considered as the low temperature increase scenario, or even as a certain cooling scenario [96]. MRI-ESM2-0 is considered as a low–medium temperature increase as an approximate global increase of 2 degrees Celsius [97]. One might refer to it as a “business as usual” model. Lastly, IPSL-CM6A-LR is considered as a medium–high increase in temperature by approximately three degrees Celsius [98]. Additionally, we cre- ated an SMD for the year 2000 with the same predictor set as the SDFs for 2100 in order to best compare current and predicted future distribution ranges.
2.4. MaxEnt
For this study, we used the Maximum Entropy software MaxEnt (https://biodiversi tyinformatics.amnh.org/open_source/maxent/) (accessed on 1 June 2022) version 3.4.4 to create the SDMs and SDFs (following Machine Learning approaches) (see [99] and references within). A thorough description of the workflow for MaxEnt SDMs can be found in Chapter 3 (Steiner and Huettmann, in-press) [8]. In coarse, the data must first be compiled, cleaned up, and standardized before they can be used for SDMs in MaxEnt. Once the models have been created with MaxEnt, their visualization has been improved and enriched by MS using ArcGIS Pro (version 2.7) (https://pro.arcgis.com/en/pro-app/ 2.8/get-started/download-arcgis-pro.htm) (accessed on 1 June 2022), and Open-Source GIS—QGIS (versions 3.10 and 3.22.7) (https://qgis.org/en/site/forusers/download.html) (accessed on 1 June 2022). For these models, we have used the standard settings for Maxent; this includes the number of iterations set to 500, and maximum number of background points set to 10,000. Additionally, for all other settings we have used the default option, which includes the feature selection, where we used “Auto Features”, including “Hinge Features”, “Product Features”, “Quadratic Features”, and “Linear Features”.
2.5. Habitat Distribution Percentage
In order to assess the spatial distribution of the models and to provide an overview of in which countries the predicted distribution occurrences can be found, we summarized the spatial information and presented it in percentage per country. This has been carried out by utilizing the QGIS tool “Zonal Statistics”, which calculates the sum of all raster cells (pixels) within a given polygon (in our case countries). Subsequently, the outcomes of this analysis have been converted into percentage per country for better interpretation.
3. Results
3.1. GPS Mapping Results
3.1.1. Species Distribution Models (SDMs)
SDMs have been created for all occurrence datasets available. They have been created for all C-datasets individually (C1, C2, and C3), and for all available data merged. The individual SDMs for all C-datasets can be found in Appendix B (Figures A6–A8). For a more holistic overview, here we present the SDMs of all available data merged. Figure 4 illustrates the predicted distribution range for the Tien Shan–Alai region.
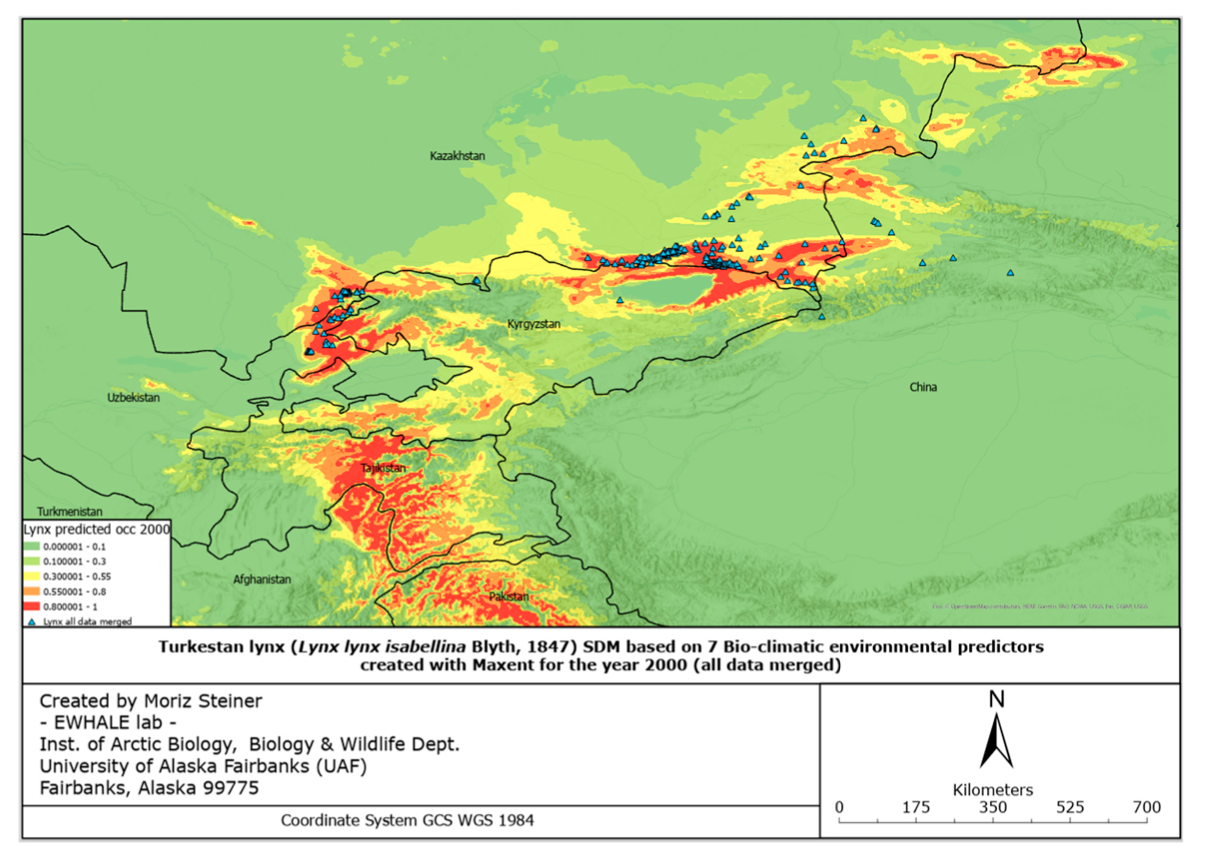
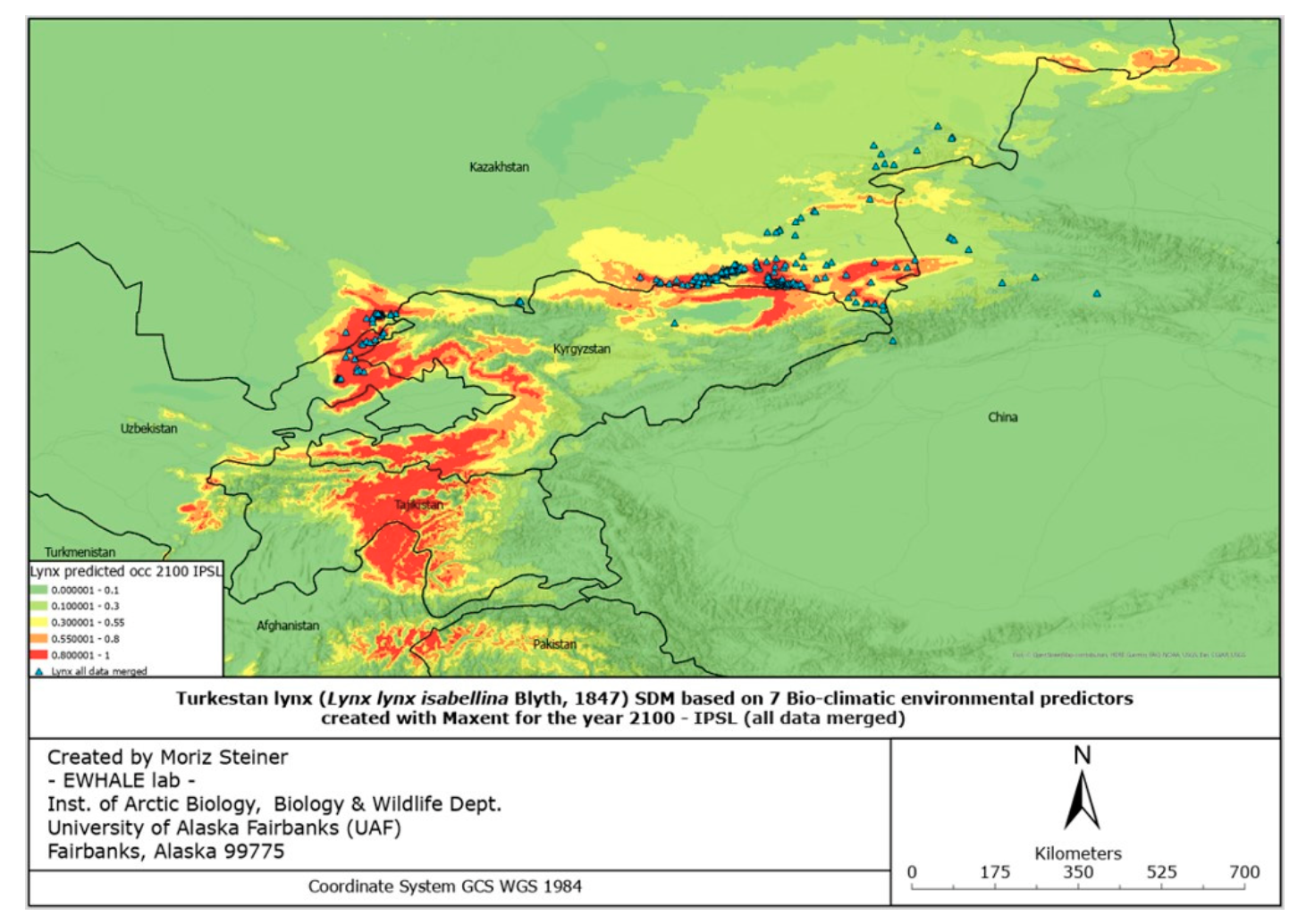
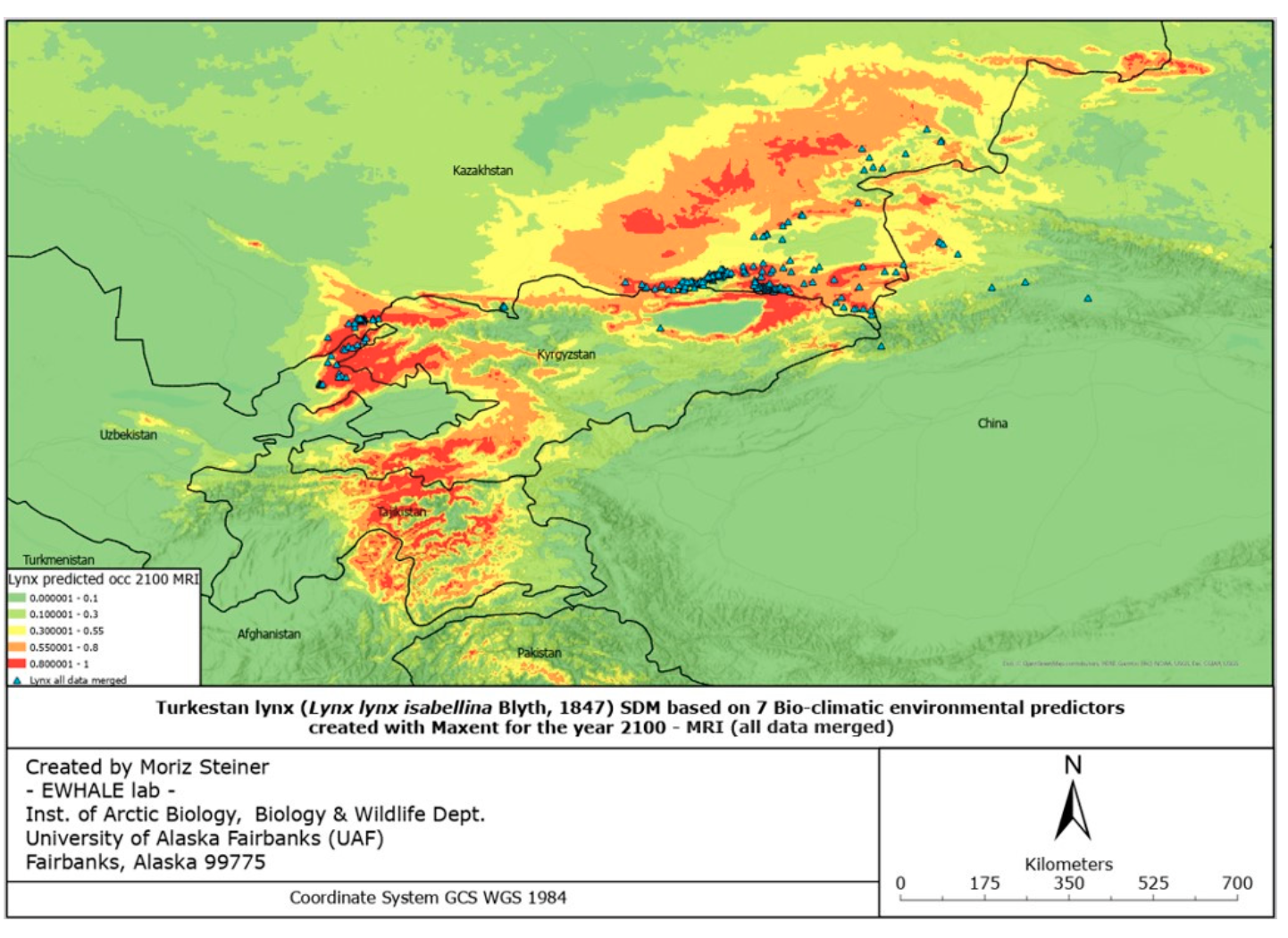
The reported Area Under the Curve (AUC) values for these SDFs were 0.982, 0.973, 0.982, and 0.984 for Figures 5-8, respectively.
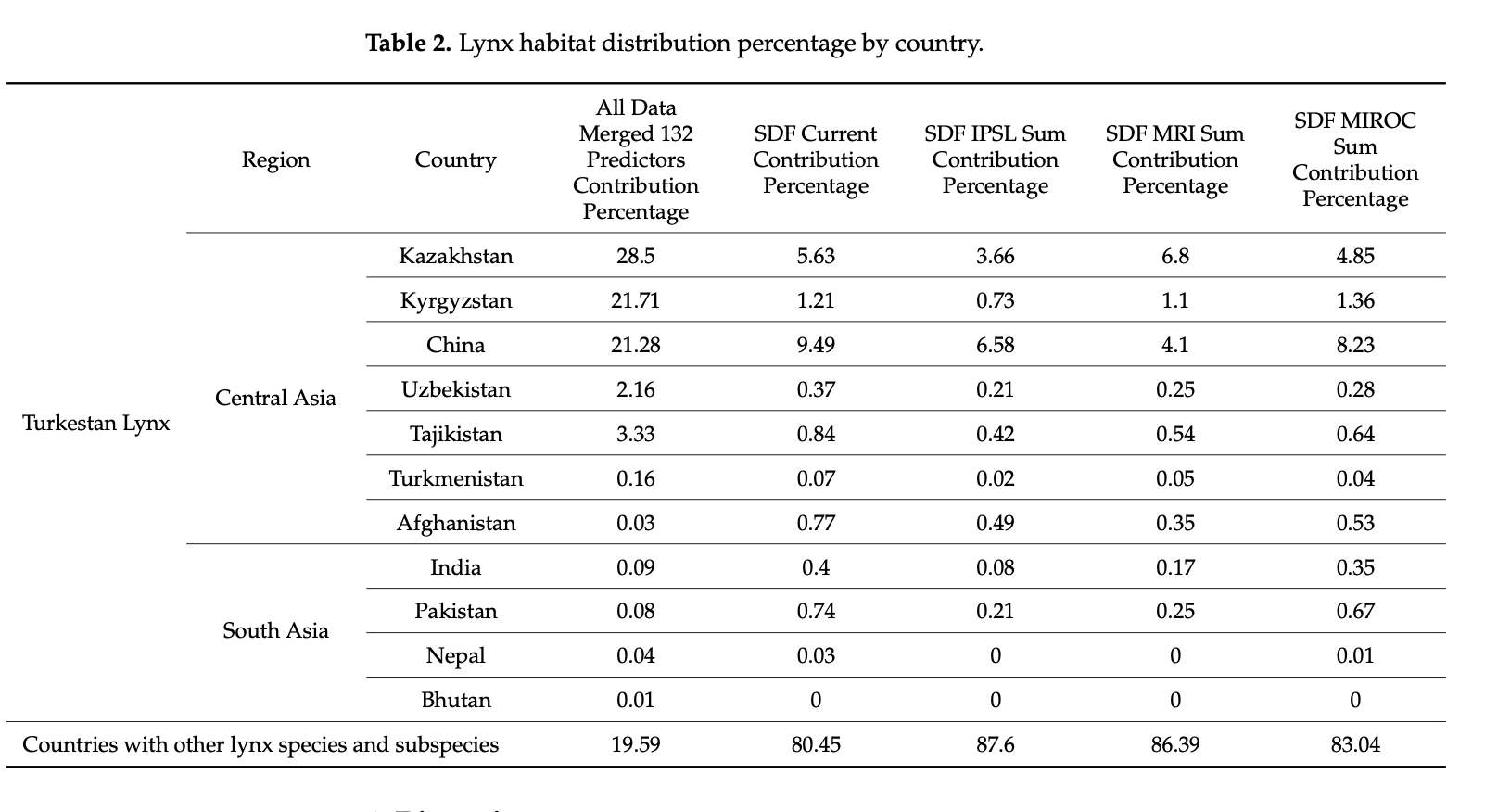
4. Discussion
4.1. Data classification
For the standardization process and usage of data obtained on lynx sightings over a large area, it is important to collect and classify the data on lynx at the local scale according to their reliability. First proposed by Molinari-Jobin et al. [92], the method to classify the data in three categories was approved and applied by a number of researchers studying lynx in mountainous conditions [91,100,101]. For this research, we also used three sets of data according to their reliability—C1 (Confirmed data), C2 (Probable occurrence) and C3 (Unconfirmed data). After evaluating the models created on these three sets of data, we noted the accuracy of all three categories of data collected laid over models. Moreover, the models of C3 data in the area of Kazakhstan and Kyrgyzstan, shown in Figures A8 and A17, seem to be highly precise and even more accurate compared to models of C2 data, illustrated in Figures A7 and A13, in the aforementioned countries. Notedly, models calculated on the C2 and C3 data do not provide the distribution information of lynx in China. As we did not obtain the C2 and C3 data from Xinjiang and the country has a different set of environmental predictors classification, the extrapolation did not cover the Xinjiang Tien Shan area for our distribution models. The accurate models created based on combined data provide the necessary insight into the predicted current and future lynx habitat distribution and dispersal.
4.2. Notes on the Turkestan Lynx’s Taxonomic Status
For the study area of this manuscript, we excluded the Altai region (N 48◦45′–51◦55′, E 83◦05′–90◦35′), located in the border region of Kazakhstan, Russia, Mongolia and China. This is due to the unclear taxonomical position of the Turkestan and Altai lynx (Lynx lynx wardi Lydekker, 1904) and where the border between the two subspecies is located in the region. According to our own observations, the differences in physical characteristics of the lynx inhabiting the Altai mountains and Zhetisu Alatau–Tien Shan mountains are distinct, with the Altai lynx being larger in size than the Turkestan lynx. Still, we included the Saur (N 47◦12′, E 85◦05′) and Tarbagatai (N 47◦14′, E 82◦13′) mountain system in the SDM and SDF maps, as the Saur–Tarbagatai lynx population has less distinguishable traits from the Turkestan lynx. Yet, some researchers believe the Saur–Tarbagatai population can still be identified as the Altai lynx, as the felid in this region frequently migrates to the Altai mountains (oral commination by Starikov S.). Thus, it is legal in Kazakhstan to hunt the lynx in the hunting grounds of the Saur mountains.
It should be noted that the subspecies differentiation between the Turkestan and Altai lynx has never been specially studied. At the same time, there is an assumption about the possibility of the Altai subspecies being identical or very close to the Turkestan lynx [29], while some researchers do not classify the Altai lynx as a separate subspecies and also consider this felid to be the Turkestan lynx [102]. For conservation purposes, it is essential that we conduct special studies in this direction. In order to determine the position of the Turkestan lynx as a subspecies and possibly strengthen its conservation status, we have conducted comparative morphometric research on lynx skulls and started molecular–genetic research, the results of which might help us to gain insight into the taxonomic position of the lynx in the region.
4.3. Analysis of Present Distribution
Considering the increasing ecological challenges for the Turkestan lynx populations such as habitat loss and fragmentation, mostly caused by overgrazing, deforestation, prey base depletion, infrastructure development, unregulated tourism, poaching, and retaliatory killings by herders [69,103], the evaluation of the elusive felid’s distribution and suitable habitats holds essential value for the creation of conservation strategies. Furthermore, assessment of the potential current lynx distribution and predicted dispersal according to a changing climate with the subsequent protection measures will also lead to the conservation of important habitats for other plant and animal species. In the northwest part of Turkestan lynx’s global range, where there has been no prior specialized and large-scale research conducted, we used our data and survey occurrence records of various lynx populations and created the first highly predictive habitat distribution models for the lynx in the region. For the current and predicted distribution forecast models, we relied on 132 environmental and 7 bio-climatic predictors, respectively. Thus, the models based on the predictors mentioned, illustrated in Figures 4 and 5, provide an ostensive characterization of present favorable lynx habitat that will accelerate the application of conservation management to areas crucial to the Turkestan lynx.
Figure 4 shows the model based on all lynx observation records obtained, combined with 132 environmental predictors, where we can visually see that lynx sightings fall within regions that our model confirms to be highly potential habitats. In particular, these regions include the Northern Tien Shan and Zhetisu Alatau areas in Kazakhstan, and the Western Tien Shan area in the border of Kazakhstan and Uzbekistan.
Notwithstanding the lack of spatial data from other Tien Shan ridges in Kyrgyzstan and China and their absence from the Western Tien Shan and Alai ridges in Tajikistan due to the fact that we did not collect data from this country, our model suggests that the distribution of lynx populations could include suitable areas in those regions as well. In particular, in Kyrgyzstan, the model presents the most propitious habitats to be located in the Ile Alatau and Kungei Alatau north to the Issyk Kul in the Northern Tien Shan, and especially in the Terskei Alatau east and south of Issyk Kul between the Northern and Inner Tien Shan, as well as in the Talas Alatau, Chatkal and Koksu ranges in the Western Tien Shan and the Fergana range between Western and Inner Tien Shan. In China, the model shows that the most highly favorable habitat is located in the Kalajun area of Northern Tien Shan in Xinjiang, near the Tekes River’s mouth. In Tajikistan, the average level of predicted occurrence is estimated to be in the Alai range at the upper stream of Zeravshan River, near the Komarou Nature Refuge.
4.4. Analysis of Predicted Movements (SDF)
The figures of the SDF models, based on seven bio-climatic predictors, indicate that the highest predicted distribution index of the Turkestan lynx, according to the current distribution, can be found in the following areas: the Ile Alatau, Kungei Alatau, Terskei Alatau and Uzynkara ranges in the Almaty region (Kazakhstan); in the Ile Alatau and Terskei Alatau ranges in the Issyk-Kul region (Kyrgyzstan); in the Ugam, Pskem and Chatkal ranges in the Tashkent region (Uzbekistan) and Jalal-Abad region (Kyrgyzstan); and Talas Alatau range in the Turkistan region (Kazakhstan). Additional hotspots can be found in the central part of Tajikistan, the northeastern part of Afghanistan, and the far north of Pakistan. Inspecting Figure 6, it can be identified that the described hotspots seem to increase towards the south-western distribution (near Tajikistan and the far western part of Tien Shan), and also near Almaty. However, the distribution seems to decrease in the northern parts (near Zhetisu Alatau (Kazakhstan)) and in the very southern parts (near Pakistan). Inspecting Figure 7, and comparing it to Figure 5, it can be identified that the described hotspots seem to change following similar patterns observed for the IPSL scenario (Figure 6), with the difference being that there is a significant increase observed near the area of Taldykorgan (Kazakhstan), which is not visible for the IPSL scenario. Lastly, for the MIROC scenario, it seems that the distribution hotspot near Almaty increases and densifies in the far western part of Tien Shan. Additionally, the southern distribution seems to decrease.
Overall, it can be observed that following the IPSL scenario, the Turkestan lynx distribution is expected to densify, move northwards when following the MRI scenario, and again densify for the MIROC scenario. Details of the observed shifts in the predicted distribution ranges (likelihood of occurrence) can be seen in Table 3 below.
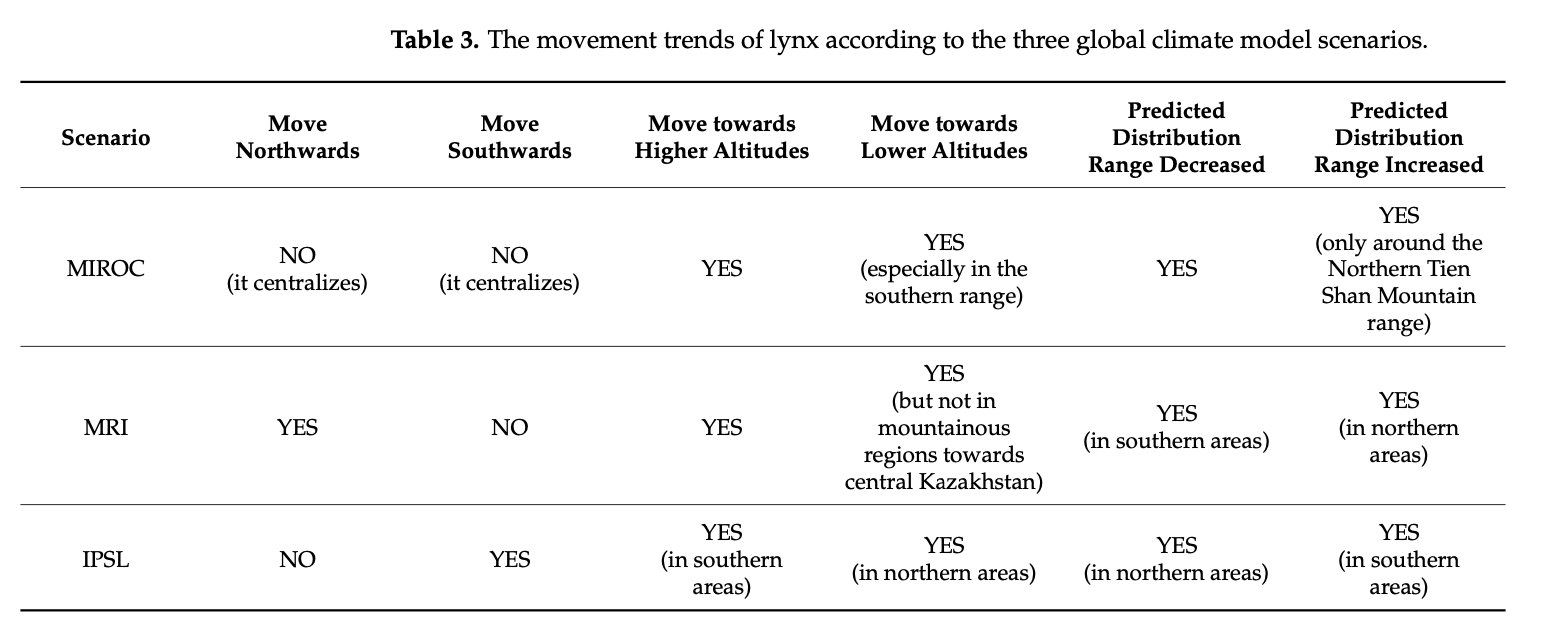
According to our SDF models based on seven bio-climatic predictors, shown in Table 2, the greatest contributions to the lynx populations are presented by China (9.49%), followed by Kazakhstan (5.63%) and Kyrgyzstan (1.21%). Kazakhstan seems to be the only country with the possible expansion of suitable habitats in the case of MRI—a possible temperature increase of approximately two degrees—but the opposite is observed with Kyrgyzstan in the case of the cooling MIROC scenario. With over 93% of the country occupied by mountainous areas [104], Tajikistan is estimated to provide 0.84% of suitable habitats for the lynx, which reduces insignificantly in cases of possible climate change. Afghanistan and Pakistan, with the presence of the Pamir and Hindu Kush mountains, follow with a similar current contribution of 0.77% and 0.74%, respectively.
Overall, in all the countries, it is observed that the lynx habitats might significantly shrink in size in the case of the IPSL scenario (significant warming scenario). Considering the calculations of the most possible MRI scenario, the predicted climate change might result in habitat reduction in all countries except Kazakhstan. This, as presented in Table 3, proves that the lynx moves northwards in the case of the MRI scenario, and we are likely to observe a significant distribution range decline in several lynx habitats with the future climate change. It must be noted that these models are “minimum-estimates”, meaning that with the low number of future predictors available, here we present a possible approximation of the future distribution range using solely seven climate predictors. We encourage future research to develop more future predictors for more robust future Species Distribution Models.
4.5. Habitat Suitability, Protected Areas and Conservation
The high percentage of favorable habitat in Kazakhstan, shown in Table 2, can be explained by a larger area of mountains in the northwest range considered for research. More precisely, the country has the largest part of the Northern Tien Shan and Zhetisu Alatau mountains. As these mountains continue in the east direction into China, the models suggest that the ridges in Xinjiang present a highly favorable environment for the lynx population and their distribution, which can be especially seen in the SDF columns of Table 2. In Kyrgyzstan, despite the lack of data used, the presence of Northern Tien Shan ridges, and a major part of the Western and Inner Tien Shan mountains, allowed the models to estimate that the country provides necessary lynx habitat; this can also be applied for the Western Tien Shan in Uzbekistan, with a high predicted lynx occurrence index in the country.
It is clear that the higher lynx occurrence indices in the study area depend on a number of environmental conditions, such as climate, land cover (coniferous forest and shrubs), access to a water source (the river and lake network), limited human disturbance, terrain (elevation and the level of annual snowfall, as well as the level of snow line), abundance of prey, and the presence of protected areas in these lynx habitats (see Figures A1–A5 and Tables A2–A6 of Appendix A). Figures A1–A5 additionally represent the omission of the models. Tables A2–A6 additionally represent the contribution percentage of the corre- sponding model, representing the “importance” of all included environmental predictors for building the model (retrieved from the HTML MaxEnt output).
The abundance of prey plays an important role in lynx stability, especially during winter [30], while extensive hunting for the prey may also result in a decline in the lynx population. During our research, we observed that in the Northern Tien Shan, during the years where the prey species, in particular, tolai hare, were declining in number, a similar tendency was noted in the lynx. In the habitats where the prey species are abundant, the lynx populations seem to be more stable, as well (Table 4).
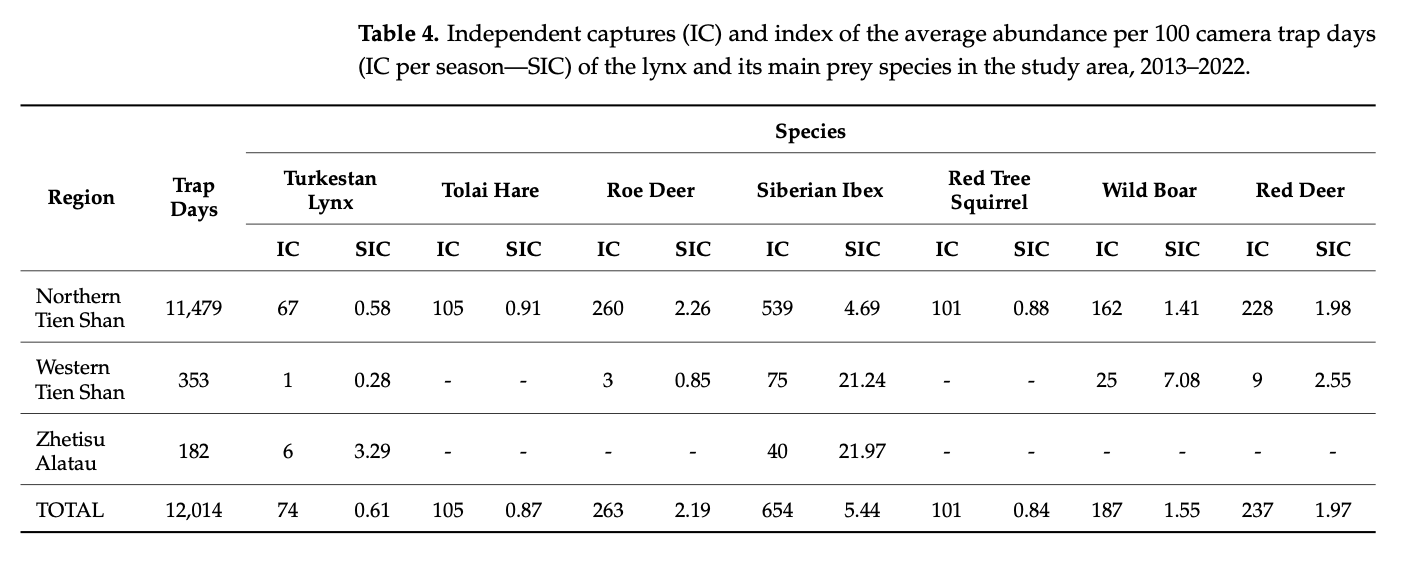
In the Northern Tien Shan, where most of our camera traps were installed, the lynx was more frequently registered by camera traps in the Kungei Alatau range compared to the Ile Alatau (on average, 0.74 and 0.44 lynx individuals per 100 trap days in Kungei Alatau and Ile Alatau ranges, respectively). In Kungei Alatau, during the period of our research, we noted a higher abundance of the tolai hare, the main lynx prey in the Tien Shan (on average, 2.34 hares per 100 trap days). In Ile Alatau, the Siberian roe deer, another key lynx prey, was frequently caught on camera (on average, 3.33 individuals per 100 trap days). In the Western Tien Shan and Zhetisu Alatau, where the camera traps were stationed for a shorter period of time, we noticed the lynx to be more frequent in the habitats with more roe deer, tolai hare (for the Western Tien Shan) and mountain hare (for the Zhetisu Alatau) present. Thus, in the territory with a relatively high abundance of prey and a smaller number of disturbance factors, a higher abundance of lynx can be traced.
Higher lynx occurrences can be noticed in habitats with protected areas within all of our study area. The level of general population stability can especially depend on the level of protection these PAs provide. In order to evaluate the contribution made by PAs for habitat suitability, we calculated the percentage of PAs laid over habitats our models estimated to be suitable for the lynx populations in the south-east of Kazakhstan, as well as the X-fold increase in predicted lynx occurrence indices of the PAs compared to the rest of Kazakhstan (as seen in Table 5 below). The calculations were made using the “Zonal statistics” tool in QGIS.
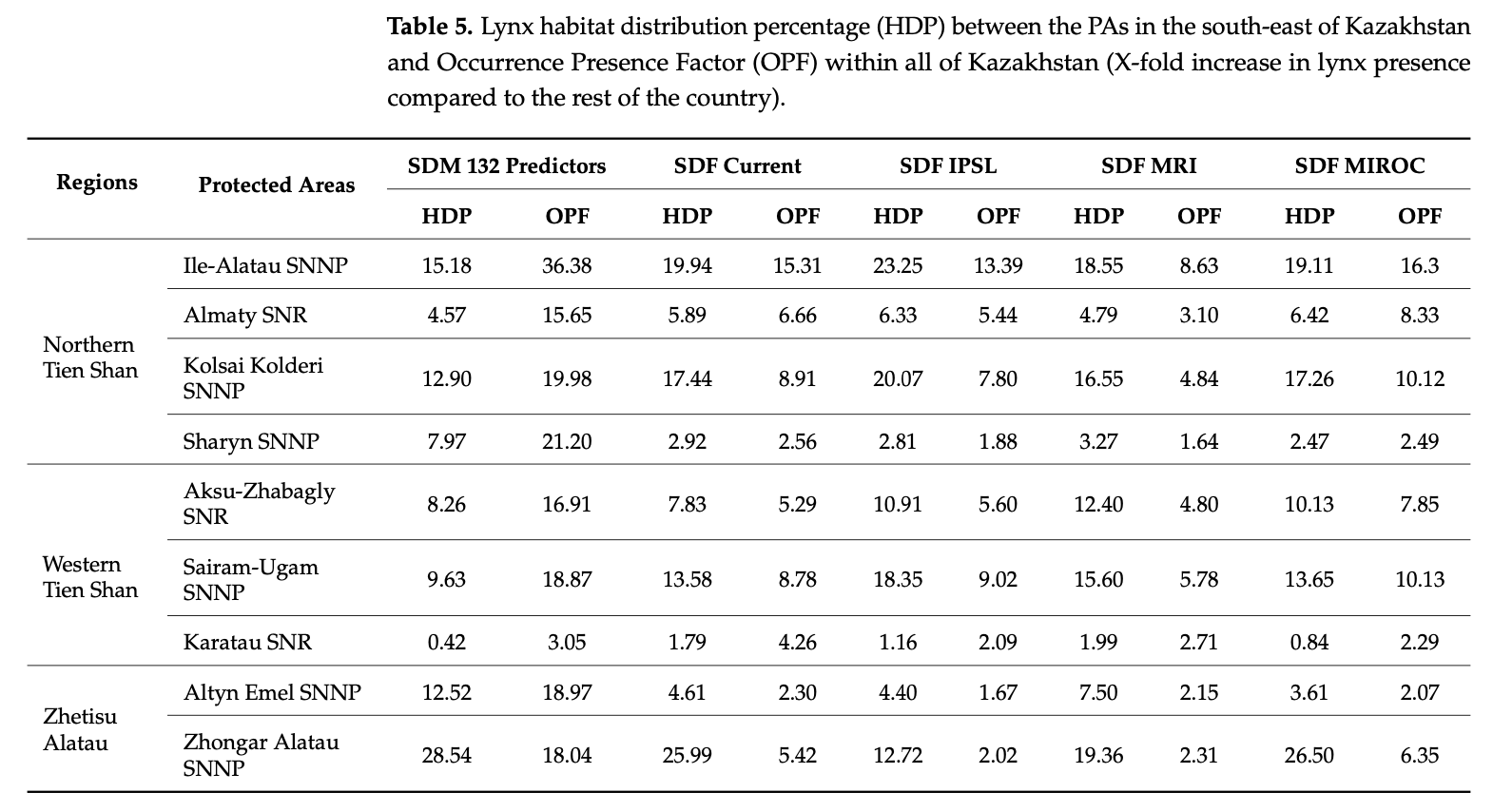
In Kazakhstan, the model based on the 132 predictors suggests that the highly favor- able habitats can be found in the Northern Tien Shan at the Ile-Alatau and Kolsai Kolderi National Parks and in the Zhetisu Alatau at the Zhongar Alatau and Altyn Emel National Parks. With the calculations of SDF models based on seven predictors, it can be seen that the Ile-Alatau, Kolsai Kolderi, and Zhongar Alatau National Parks and the Sairam-Ugam National Park in the Western Tien Shan play a significant role in conserving the lynx habi- tats in future climate change scenarios. In the case of Nature Reserves, where the level of protection is the highest, Almaty Nature Reserve provides the key habitats to the lynx populations despite having much less territory compared to the other National Parks in the Northern Tien Shan.
In China, the possible lynx habitats are protected in the Tomur Peak National Nature Reserve, Kalajun Provincal Park, Kuerdening National Nature Reserve, and Bayinbuluke National Nature Park, with our models estimating the most suitable habitats being located in the Kalajun Provincal Park area. In Kyrgyzstan, the models show that the relevant lynx habitats are located at and protected by the Chong-Kemin National Park on the Ile Alatau and Kungei Alatau ranges of Northern Tien Shan, the Besh-Aral Reserve on the Chatkal Valley of Western Tien Shan, and the Issyk-Kul and Naryn Reserves and the Saymaluu- Tash National Park on the Fergana range in the Inner Tien Shan; in the Karatal-Japyryk Nature Reserve in the Inner Tien Shan the lynx number seems to slightly increase over the years [56]. In Uzbekistan, the Ugam Chatkal Nature Park, Chatkal Nature Reserve and Ugam Chatkal Reserve in the Western Tien Shan maintain the habitats for the lynx and its prey species [67]. In Tajikistan, SDM shows the occurrences being mostly near the Komarou Nature Refuge.
As the threats to the lynx populations are still present and increasing in the mountains, the key solution for conservation of the felid and other animal and plant species, as well as their habitats, is to create new PAs in the areas where they are most necessary, as well as expand the territory of the existing PAs. In Kazakhstan, one of key countries for the lynx habitats, we recommend creation of Uzynkara Nature Reserve in the Uzynkara range of Northern Tien Shan, while in this and Terskei Alatau range there is no PA present yet. As the Ile-Alatau National Park is located in the vicinity and partially in the territory of Almaty megapolis, it is also important to increase the size of the park and strengthen its protection system.
5. Conclusions
In summary, our results describe the present distribution and future dispersal for the year 2100 of Turkestan lynx at the northernmost part of their global geographical range—in the Northern and Western Tien Shan and Zhetisu Alatau mountains. The highly predictive distribution models provide the data on suitable habitats in our study area, as well as within all 11 countries of its distribution. According to the SDM models on 132 environmental predictors and our subsequent calculations, we noted the most suitable lynx habitats to be located in Kazakhstan, followed by Kyrgyzstan and China. Based on seven bio-climatic environmental predictors, we estimate a lynx habitat reduction in most predicted future climate scenarios.
With the significant proportion of suitable habitat relevant to the lynx population located in the northwestern part of its global range, it is evident that the Northern and Western Tien Shan and Zhetisu Alatau mountains are crucial for lynx population stability and future survival. For the conservation of the lynx and other rare animals, it is essential to create new and expand existing protected areas, make a collaboration between the countries to study this felid, and create ecological corridors between the mountain regions and countries contributing to lynx distribution in Central and South Asia.
Acknowledgments: The authors express their sincere gratitude to the administration, researchers, game managers, inspectors and other employees of the Almaty Reserve, Ile-Alatau National Park, Kolsai Kolderi National Park, Sharyn National Park, Altyn Emel National Park, Aksu-Zhabagly Nature Reserve, Sairam-Ugam National Park and al-Farabi Kazakh National University, and other organizations involved for their help in the preparation and implementation of these studies. In partic- ular, from the Ile-Alatau SNNP—Head of the Department of Research and Mountain Agrobiocenosis Saltanat Userbayeva, Researcher Berik Nesipbai, Head of the Department of Protection and Regenera- tion of the Wildlife Bauyrzhan Rustemkhanuly, Rangers and Game Managers Meirzhan Kistykbayev, Sergey Strebkov, Andrey Segayev, Ulan Estemesov, Askar Kali, and others; from the Almaty SNR— Researcher Altynbek Dzhanyspayev, Rangers Zhanbolat Baimukhanov, Alexander Matvienko, and others; from the Kolsai Kolderi SNNP—Khamit Akhmetov, and others; from the Department of Biodiversity and bioresources at al-Farabi Kazakh National University—Meruyert Kurmanbayeva, Sandugash Mankibayeva, Ruslan Salmurzauly, and the late Kuandyk Saparov who has passed away during the pandemic, and who we included as a co-author of this manuscript; from the “Ecological Education” group—Head of the group Elena Udartseva; from the Wild Nature NGO—Director Svetlana Baskakova, from Wildlife Without Borders NGO—Director Dina Konysbayeva, and others.
Conflicts of Interest: The authors declare no conflict of interest.